mirror of
https://github.com/twitter/the-algorithm.git
synced 2025-01-09 02:40:42 +01:00
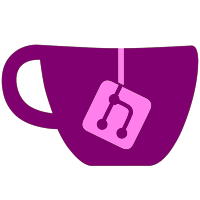
Please note we have force-pushed a new initial commit in order to remove some publicly-available Twitter user information. Note that this process may be required in the future.
1381 lines
56 KiB
Python
1381 lines
56 KiB
Python
"""
|
|
This module contains custom tensorflow metrics used at Twitter.
|
|
Its components conform to conventions used by the ``tf.metrics`` module.
|
|
|
|
"""
|
|
|
|
from collections import OrderedDict
|
|
from functools import partial
|
|
|
|
import numpy as np
|
|
import tensorboard as tb
|
|
import tensorflow.compat.v1 as tf
|
|
|
|
|
|
CLAMP_EPSILON = 0.00001
|
|
|
|
|
|
def total_weight_metric(
|
|
labels,
|
|
predictions,
|
|
weights=None,
|
|
metrics_collections=None,
|
|
updates_collections=None,
|
|
name=None):
|
|
with tf.variable_scope(name, 'total_weight', (labels, predictions, weights)):
|
|
total_weight = _metric_variable(name='total_weight', shape=[], dtype=tf.float64)
|
|
|
|
if weights is None:
|
|
weights = tf.cast(tf.size(labels), total_weight.dtype, name="default_weight")
|
|
else:
|
|
weights = tf.cast(weights, total_weight.dtype)
|
|
|
|
# add up the weights to get total weight of the eval set
|
|
update_total_weight = tf.assign_add(total_weight, tf.reduce_sum(weights), name="update_op")
|
|
|
|
value_op = tf.identity(total_weight)
|
|
update_op = tf.identity(update_total_weight)
|
|
|
|
if metrics_collections:
|
|
tf.add_to_collections(metrics_collections, value_op)
|
|
|
|
if updates_collections:
|
|
tf.add_to_collections(updates_collections, update_op)
|
|
|
|
return value_op, update_op
|
|
|
|
|
|
def num_samples_metric(
|
|
labels,
|
|
predictions,
|
|
weights=None,
|
|
metrics_collections=None,
|
|
updates_collections=None,
|
|
name=None):
|
|
with tf.variable_scope(name, 'num_samples', (labels, predictions, weights)):
|
|
num_samples = _metric_variable(name='num_samples', shape=[], dtype=tf.float64)
|
|
update_num_samples = tf.assign_add(num_samples, tf.cast(tf.size(labels), num_samples.dtype), name="update_op")
|
|
|
|
value_op = tf.identity(num_samples)
|
|
update_op = tf.identity(update_num_samples)
|
|
|
|
if metrics_collections:
|
|
tf.add_to_collections(metrics_collections, value_op)
|
|
|
|
if updates_collections:
|
|
tf.add_to_collections(updates_collections, update_op)
|
|
|
|
return value_op, update_op
|
|
|
|
|
|
def ctr(labels, predictions,
|
|
weights=None,
|
|
metrics_collections=None,
|
|
updates_collections=None,
|
|
name=None):
|
|
# pylint: disable=unused-argument
|
|
"""
|
|
Compute the weighted average positive sample ratio based on labels
|
|
(i.e. weighted average percentage of positive labels).
|
|
The name `ctr` (click-through-rate) is from legacy.
|
|
|
|
Args:
|
|
labels: the ground truth value.
|
|
predictions: the predicted values, whose shape must match labels. Ignored for CTR computation.
|
|
weights: optional weights, whose shape must match labels . Weight is 1 if not set.
|
|
metrics_collections: optional list of collections to add this metric into.
|
|
updates_collections: optional list of collections to add the associated update_op into.
|
|
name: an optional variable_scope name.
|
|
|
|
Return:
|
|
ctr: A `Tensor` representing positive sample ratio.
|
|
update_op: A update operation used to accumulate data into this metric.
|
|
"""
|
|
return tf.metrics.mean(
|
|
values=labels,
|
|
weights=weights,
|
|
metrics_collections=metrics_collections,
|
|
updates_collections=updates_collections,
|
|
name=name)
|
|
|
|
|
|
def predicted_ctr(labels, predictions,
|
|
weights=None,
|
|
metrics_collections=None,
|
|
updates_collections=None,
|
|
name=None):
|
|
# pylint: disable=unused-argument
|
|
"""
|
|
Compute the weighted average positive ratio based on predictions,
|
|
(i.e. weighted averaged predicted positive probability).
|
|
The name `ctr` (click-through-rate) is from legacy.
|
|
|
|
Args:
|
|
labels: the ground truth value.
|
|
predictions: the predicted values, whose shape must match labels. Ignored for CTR computation.
|
|
weights: optional weights, whose shape must match labels . Weight is 1 if not set.
|
|
metrics_collections: optional list of collections to add this metric into.
|
|
updates_collections: optional list of collections to add the associated update_op into.
|
|
name: an optional variable_scope name.
|
|
|
|
Return:
|
|
predicted_ctr: A `Tensor` representing the predicted positive ratio.
|
|
update_op: A update operation used to accumulate data into this metric.
|
|
"""
|
|
return tf.metrics.mean(
|
|
values=predictions,
|
|
weights=weights,
|
|
metrics_collections=metrics_collections,
|
|
updates_collections=updates_collections,
|
|
name=name)
|
|
|
|
|
|
def prediction_std_dev(labels, predictions,
|
|
weights=None,
|
|
metrics_collections=None,
|
|
updates_collections=None,
|
|
name=None):
|
|
"""
|
|
Compute the weighted standard deviation of the predictions.
|
|
Note - this is not a confidence interval metric.
|
|
|
|
Args:
|
|
labels: the ground truth value.
|
|
predictions: the predicted values, whose shape must match labels. Ignored for CTR computation.
|
|
weights: optional weights, whose shape must match labels . Weight is 1 if not set.
|
|
metrics_collections: optional list of collections to add this metric into.
|
|
updates_collections: optional list of collections to add the associated update_op into.
|
|
name: an optional variable_scope name.
|
|
|
|
Return:
|
|
metric value: A `Tensor` representing the value of the metric on the data accumulated so far.
|
|
update_op: A update operation used to accumulate data into this metric.
|
|
"""
|
|
with tf.variable_scope(name, 'pred_std_dev', (labels, predictions, weights)):
|
|
labels = tf.cast(labels, tf.float64)
|
|
predictions = tf.cast(predictions, tf.float64)
|
|
|
|
if weights is None:
|
|
weights = tf.ones(shape=tf.shape(labels), dtype=tf.float64, name="default_weight")
|
|
else:
|
|
weights = tf.cast(weights, tf.float64)
|
|
|
|
# State kept during streaming of examples
|
|
total_weighted_preds = _metric_variable(
|
|
name='total_weighted_preds', shape=[], dtype=tf.float64)
|
|
total_weighted_preds_sq = _metric_variable(
|
|
name='total_weighted_preds_sq', shape=[], dtype=tf.float64)
|
|
total_weights = _metric_variable(
|
|
name='total_weights', shape=[], dtype=tf.float64)
|
|
|
|
# Update state
|
|
update_total_weighted_preds = tf.assign_add(total_weighted_preds, tf.reduce_sum(weights * predictions))
|
|
update_total_weighted_preds_sq = tf.assign_add(total_weighted_preds_sq, tf.reduce_sum(weights * predictions * predictions))
|
|
update_total_weights = tf.assign_add(total_weights, tf.reduce_sum(weights))
|
|
|
|
# Compute output
|
|
def compute_output(tot_w, tot_wp, tot_wpp):
|
|
return tf.math.sqrt(tot_wpp / tot_w - (tot_wp / tot_w) ** 2)
|
|
std_dev_est = compute_output(total_weights, total_weighted_preds, total_weighted_preds_sq)
|
|
update_std_dev_est = compute_output(update_total_weights, update_total_weighted_preds, update_total_weighted_preds_sq)
|
|
|
|
if metrics_collections:
|
|
tf.add_to_collections(metrics_collections, std_dev_est)
|
|
|
|
if updates_collections:
|
|
tf.add_to_collections(updates_collections, update_std_dev_est)
|
|
|
|
return std_dev_est, update_std_dev_est
|
|
|
|
|
|
def _get_arce_predictions(predictions, weights, label_weighted, labels,
|
|
up_weight, deprecated_rce,
|
|
total_positive, update_total_positive):
|
|
"""
|
|
Returns the ARCE predictions, total_positive, update_total_positive and weights
|
|
used by the rest of the twml.metrics.rce metric computation.
|
|
"""
|
|
predictions_weighted = tf.multiply(predictions, weights, name="weighted_preds")
|
|
label_weighted_comp = tf.subtract(tf.reduce_sum(weights), tf.reduce_sum(label_weighted))
|
|
pred_weight_comp = tf.subtract(tf.reduce_sum(weights), tf.reduce_sum(predictions_weighted))
|
|
normalizer_comp = label_weighted_comp / pred_weight_comp
|
|
|
|
if up_weight is False:
|
|
total_positive_unweighted = _metric_variable(
|
|
name='total_positive_unweighted', shape=[], dtype=tf.float32)
|
|
|
|
update_total_positive_unweighted = tf.assign_add(
|
|
total_positive_unweighted, tf.reduce_sum(labels),
|
|
name="total_positive_unweighted_update")
|
|
|
|
if deprecated_rce:
|
|
normalizer = tf.reduce_sum(labels) / tf.reduce_sum(label_weighted)
|
|
else:
|
|
# sum of labels / sum of weighted labels
|
|
normalizer = update_total_positive_unweighted / update_total_positive
|
|
|
|
label_comp = tf.subtract(tf.to_float(tf.size(labels)), tf.reduce_sum(labels))
|
|
normalizer_comp = label_comp / label_weighted_comp
|
|
|
|
# note that up_weight=True changes these for the rest of the twml.metric.rce computation
|
|
weights = tf.ones(shape=tf.shape(labels), dtype=tf.float32, name="default_weight")
|
|
total_positive = total_positive_unweighted
|
|
update_total_positive = update_total_positive_unweighted
|
|
else:
|
|
if deprecated_rce:
|
|
normalizer = tf.reduce_sum(label_weighted) / tf.reduce_sum(predictions_weighted)
|
|
else:
|
|
# normalizer used for NRCE (and ARCE with up_weight=True)
|
|
total_prediction = _metric_variable(name='total_prediction', shape=[], dtype=tf.float32)
|
|
|
|
# update the variable holding the sum of weighted predictions
|
|
update_total_prediction = tf.assign_add(
|
|
total_prediction, tf.reduce_sum(predictions_weighted), name="total_prediction_update")
|
|
|
|
# this used to be tf.reduce_sum(label_weighted) / tf.reduce_sum(predictions_weighted)
|
|
# but it measure normalizer over batch was too flawed an approximation.
|
|
normalizer = update_total_positive / update_total_prediction
|
|
|
|
pred_comp = tf.subtract(tf.ones(shape=tf.shape(labels), dtype=tf.float32), predictions)
|
|
pred_comp_norm = tf.multiply(pred_comp, normalizer_comp, name="normalized_predictions_comp")
|
|
pred_num = tf.multiply(predictions, normalizer, name="normalized_pred_numerator")
|
|
pred_denom = tf.add(pred_num, pred_comp_norm, name="normalized_pred_denominator")
|
|
predictions = pred_num / pred_denom
|
|
|
|
return predictions, total_positive, update_total_positive, weights
|
|
|
|
|
|
def rce(labels, predictions,
|
|
weights=None,
|
|
normalize=False,
|
|
arce=False,
|
|
up_weight=True,
|
|
metrics_collections=None,
|
|
updates_collections=None,
|
|
name=None,
|
|
deprecated_rce=False):
|
|
"""
|
|
Compute the relative cross entropy (RCE).
|
|
The RCE is a relative measurement compared to the baseline model's performance.
|
|
The baseline model always predicts average click-through-rate (CTR).
|
|
The RCE measures, in percentage, how much better the predictions are, compared
|
|
to the baseline model, in terms of cross entropy loss.
|
|
|
|
y = label; p = prediction;
|
|
binary cross entropy = y * log(p) + (1-y) * log(1-p)
|
|
|
|
Args:
|
|
labels:
|
|
the ground true value.
|
|
predictions:
|
|
the predicted values, whose shape must match labels.
|
|
weights:
|
|
optional weights, whose shape must match labels . Weight is 1 if not set.
|
|
normalize:
|
|
if set to true, produce NRCEs used at Twitter. (normalize preds by weights first)
|
|
NOTE: if you don't understand what NRCE is, please don't use it.
|
|
arce:
|
|
if set to true, produces `ARCE <http://go/arce>`_.
|
|
This can only be activated if `normalize=True`.
|
|
up_weight:
|
|
if set to true, produces arce in the up_weighted space (considers CTR after up_weighting
|
|
data), while False gives arce in the original space (only considers CTR before up_weighting).
|
|
In the actual version, this flag can only be activated if arce is True.
|
|
Notice that the actual version of NRCE corresponds to up_weight=True.
|
|
metrics_collections:
|
|
optional list of collections to add this metric into.
|
|
updates_collections:
|
|
optional list of collections to add the associated update_op into.
|
|
name:
|
|
an optional variable_scope name.
|
|
deprecated_rce:
|
|
enables the previous NRCE/ARCE calculations which calculated some label metrics
|
|
on the batch instead of on all batches seen so far. Note that the older metric
|
|
calculation is less stable, especially for smaller batch sizes. You should probably
|
|
never have to set this to True.
|
|
|
|
Return:
|
|
rce_value:
|
|
A ``Tensor`` representing the RCE.
|
|
update_op:
|
|
A update operation used to accumulate data into this metric.
|
|
|
|
.. note:: Must have at least 1 positive and 1 negative sample accumulated,
|
|
or RCE will come out as NaN.
|
|
"""
|
|
with tf.variable_scope(name, 'rce', (labels, predictions, weights)):
|
|
labels = tf.to_float(labels, name="label_to_float")
|
|
predictions = tf.to_float(predictions, name="predictions_to_float")
|
|
|
|
if weights is None:
|
|
weights = tf.ones(shape=tf.shape(labels), dtype=tf.float32, name="default_weight")
|
|
else:
|
|
weights = tf.to_float(weights, name="weight_to_float")
|
|
|
|
total_positive = _metric_variable(name='total_positive', shape=[], dtype=tf.float32)
|
|
total_loss = _metric_variable(name='total_loss', shape=[], dtype=tf.float32)
|
|
total_weight = _metric_variable(name='total_weight', shape=[], dtype=tf.float32)
|
|
|
|
label_weighted = tf.multiply(labels, weights, name="weighted_label")
|
|
|
|
update_total_positive = tf.assign_add(
|
|
total_positive, tf.reduce_sum(label_weighted), name="total_pos_update")
|
|
|
|
if arce:
|
|
if normalize is False:
|
|
raise ValueError('This configuration of parameters is not actually allowed')
|
|
|
|
predictions, total_positive, update_total_positive, weights = _get_arce_predictions(
|
|
predictions=predictions, weights=weights, deprecated_rce=deprecated_rce,
|
|
label_weighted=label_weighted, labels=labels, up_weight=up_weight,
|
|
total_positive=total_positive, update_total_positive=update_total_positive)
|
|
|
|
elif normalize:
|
|
predictions_weighted = tf.multiply(predictions, weights, name="weighted_preds")
|
|
|
|
if deprecated_rce:
|
|
normalizer = tf.reduce_sum(label_weighted) / tf.reduce_sum(predictions_weighted)
|
|
else:
|
|
total_prediction = _metric_variable(name='total_prediction', shape=[], dtype=tf.float32)
|
|
|
|
# update the variable holding the sum of weighted predictions
|
|
update_total_prediction = tf.assign_add(
|
|
total_prediction, tf.reduce_sum(predictions_weighted), name="total_prediction_update")
|
|
|
|
# this used to be tf.reduce_sum(label_weighted) / tf.reduce_sum(predictions_weighted)
|
|
# but it measure normalizer over batch was too flawed an approximation.
|
|
normalizer = update_total_positive / update_total_prediction
|
|
|
|
# NRCE
|
|
predictions = tf.multiply(predictions, normalizer, name="normalized_predictions")
|
|
|
|
# clamp predictions to keep log(p) stable
|
|
clip_p = tf.clip_by_value(predictions, CLAMP_EPSILON, 1.0 - CLAMP_EPSILON, name="clip_p")
|
|
logloss = _binary_cross_entropy(pred=clip_p, target=labels, name="logloss")
|
|
|
|
logloss_weighted = tf.multiply(logloss, weights, name="weighted_logloss")
|
|
|
|
update_total_loss = tf.assign_add(
|
|
total_loss, tf.reduce_sum(logloss_weighted), name="total_loss_update")
|
|
update_total_weight = tf.assign_add(
|
|
total_weight, tf.reduce_sum(weights), name="total_weight_update")
|
|
|
|
# metric value retrieval subgraph
|
|
ctr1 = tf.truediv(total_positive, total_weight, name="ctr")
|
|
# Note: we don't have to keep running averages for computing baseline CE. Because the prediction
|
|
# is constant for every sample, we can simplify it to the formula below.
|
|
baseline_ce = _binary_cross_entropy(pred=ctr1, target=ctr1, name="baseline_ce")
|
|
pred_ce = tf.truediv(total_loss, total_weight, name="pred_ce")
|
|
|
|
rce_t = tf.multiply(
|
|
1.0 - tf.truediv(pred_ce, baseline_ce),
|
|
100,
|
|
name="rce")
|
|
|
|
# metric update subgraph
|
|
ctr2 = tf.truediv(update_total_positive, update_total_weight, name="ctr_update")
|
|
# Note: we don't have to keep running averages for computing baseline CE. Because the prediction
|
|
# is constant for every sample, we can simplify it to the formula below.
|
|
baseline_ce2 = _binary_cross_entropy(pred=ctr2, target=ctr2, name="baseline_ce_update")
|
|
pred_ce2 = tf.truediv(update_total_loss, update_total_weight, name="pred_ce_update")
|
|
|
|
update_op = tf.multiply(
|
|
1.0 - tf.truediv(pred_ce2, baseline_ce2),
|
|
100,
|
|
name="update_op")
|
|
|
|
if metrics_collections:
|
|
tf.add_to_collections(metrics_collections, rce_t)
|
|
|
|
if updates_collections:
|
|
tf.add_to_collections(updates_collections, update_op)
|
|
|
|
return rce_t, update_op
|
|
|
|
|
|
def ce(p_true, p_est=None):
|
|
if p_est is None:
|
|
p_est = p_true
|
|
return _binary_cross_entropy(pred=p_est, target=p_true, name=None)
|
|
|
|
|
|
def rce_transform(outputs, labels, weights):
|
|
'''
|
|
Construct an OrderedDict of quantities to aggregate over eval batches
|
|
outputs, labels, weights are TensorFlow tensors, and are assumed to
|
|
be of shape [N] for batch_size = N
|
|
Each entry in the output OrderedDict should also be of shape [N]
|
|
'''
|
|
out_vals = OrderedDict()
|
|
out_vals['weighted_loss'] = weights * ce(p_true=labels, p_est=outputs)
|
|
out_vals['weighted_labels'] = labels * weights
|
|
out_vals['weight'] = weights
|
|
return out_vals
|
|
|
|
|
|
def rce_metric(aggregates):
|
|
'''
|
|
input ``aggregates`` is an OrderedDict with the same keys as those created
|
|
by rce_transform(). The dict values are the aggregates (reduce_sum)
|
|
of the values produced by rce_transform(), and should be scalars.
|
|
output is the value of RCE
|
|
'''
|
|
# cummulative weighted loss of model predictions
|
|
total_weighted_loss = aggregates['weighted_loss']
|
|
total_weighted_labels = aggregates['weighted_labels']
|
|
total_weight = aggregates['weight']
|
|
|
|
model_average_loss = total_weighted_loss / total_weight
|
|
baseline_average_loss = ce(total_weighted_labels / total_weight)
|
|
return 100.0 * (1 - model_average_loss / baseline_average_loss)
|
|
|
|
|
|
def metric_std_err(labels, predictions,
|
|
weights=None,
|
|
transform=rce_transform, metric=rce_metric,
|
|
metrics_collections=None,
|
|
updates_collections=None,
|
|
name='rce_std_err'):
|
|
"""
|
|
Compute the weighted standard error of the RCE metric on this eval set.
|
|
This can be used for confidence intervals and unpaired hypothesis tests.
|
|
|
|
Args:
|
|
labels: the ground truth value.
|
|
predictions: the predicted values, whose shape must match labels.
|
|
weights: optional weights, whose shape must match labels . Weight is 1 if not set.
|
|
transform: a function of the following form:
|
|
|
|
.. code-block:: python
|
|
|
|
def transform(outputs, labels, weights):
|
|
out_vals = OrderedDict()
|
|
...
|
|
return out_vals
|
|
|
|
where outputs, labels, and weights are all tensors of shape [eval_batch_size].
|
|
The returned OrderedDict() should have values that are tensors of shape [eval_batch_size].
|
|
These will be aggregated across many batches in the eval dataset, to produce
|
|
one scalar value per key of out_vals.
|
|
metric: a function of the following form
|
|
|
|
.. code-block:: python
|
|
|
|
def metric(aggregates):
|
|
...
|
|
return metric_value
|
|
|
|
where aggregates is an OrderedDict() having the same keys created by transform().
|
|
Each of the corresponding dict values is the reduce_sum of the values produced by
|
|
transform(), and is a TF scalar. The return value should be a scalar representing
|
|
the value of the desired metric.
|
|
metrics_collections: optional list of collections to add this metric into.
|
|
updates_collections: optional list of collections to add the associated update_op into.
|
|
name: an optional variable_scope name.
|
|
|
|
Return:
|
|
metric value: A `Tensor` representing the value of the metric on the data accumulated so far.
|
|
update_op: A update operation used to accumulate data into this metric.
|
|
"""
|
|
with tf.variable_scope(name, 'metric_std_err', (labels, predictions, weights)):
|
|
labels = tf.cast(labels, tf.float64)
|
|
predictions = tf.cast(predictions, tf.float64)
|
|
|
|
if weights is None:
|
|
weights = tf.ones_like(labels, dtype=tf.float64, name="default_weight")
|
|
else:
|
|
weights = tf.cast(weights, tf.float64)
|
|
|
|
labels = tf.reshape(labels, [-1])
|
|
predictions = tf.reshape(predictions, [-1])
|
|
predictions = tf.clip_by_value(predictions, CLAMP_EPSILON, 1.0 - CLAMP_EPSILON, name="clip_p")
|
|
weights = tf.reshape(weights, [-1])
|
|
|
|
# first apply the supplied transform function to the output, label, weight data
|
|
# returns an OrderedDict of 1xN tensors for N input samples
|
|
# for each sample, compute f = transform(pred, l, w)
|
|
transformed = transform(predictions, labels, weights)
|
|
|
|
# we track 3 types of aggregate information
|
|
# 1. total number of samples
|
|
# 2. aggregated transformed samples (moment1), i.e. sum(f)
|
|
# 3. aggregated crosses of transformed samples (moment2), i.e. sum(f*f^T)
|
|
|
|
# count total number of samples
|
|
sample_count = _metric_variable(
|
|
name='sample_count', shape=[], dtype=tf.int64)
|
|
update_sample_count = tf.assign_add(sample_count, tf.size(labels, out_type=sample_count.dtype))
|
|
|
|
# compose the ordered dict into a single vector
|
|
# so f can be treated as a single column vector rather than a collection of scalars
|
|
N = len(transformed)
|
|
transformed_vec = tf.stack(list(transformed.values()), axis=1)
|
|
|
|
# compute and update transformed samples (1st order statistics)
|
|
# i.e. accumulate f into F as F += sum(f)
|
|
aggregates_1 = _metric_variable(
|
|
name='aggregates_1', shape=[N], dtype=tf.float64)
|
|
update_aggregates_1 = tf.assign_add(aggregates_1, tf.reduce_sum(transformed_vec, axis=0))
|
|
|
|
# compute and update crossed transformed samples (2nd order statistics)
|
|
# i.e. accumulate f*f^T into F2 as F2 += sum(f*transpose(f))
|
|
aggregates_2 = _metric_variable(
|
|
name='aggregates_2', shape=[N, N], dtype=tf.float64)
|
|
moment_2_temp = (
|
|
tf.reshape(transformed_vec, shape=[-1, N, 1])
|
|
* tf.reshape(transformed_vec, shape=[-1, 1, N])
|
|
)
|
|
update_aggregates_2 = tf.assign_add(aggregates_2, tf.reduce_sum(moment_2_temp, axis=0))
|
|
|
|
def compute_output(agg_1, agg_2, samp_cnt):
|
|
# decompose the aggregates back into a dict to pass to the user-supplied metric fn
|
|
aggregates_dict = OrderedDict()
|
|
for i, key in enumerate(transformed.keys()):
|
|
aggregates_dict[key] = agg_1[i]
|
|
|
|
metric_value = metric(aggregates_dict)
|
|
|
|
# derivative of metric with respect to the 1st order aggregates
|
|
# i.e. d M(agg1) / d agg1
|
|
metric_prime = tf.gradients(metric_value, agg_1, stop_gradients=agg_1)
|
|
|
|
# estimated covariance of agg_1
|
|
# cov(F) = sum(f*f^T) - (sum(f) * sum(f)^T) / N
|
|
# = agg_2 - (agg_1 * agg_1^T) / N
|
|
N_covariance_estimate = agg_2 - (
|
|
tf.reshape(agg_1, shape=[-1, 1])
|
|
@ tf.reshape(agg_1, shape=[1, -1])
|
|
/ tf.cast(samp_cnt, dtype=tf.float64)
|
|
)
|
|
|
|
# push N_covariance_estimate through a linearization of metric around agg_1
|
|
# metric var = transpose(d M(agg1) / d agg1) * cov(F) * (d M(agg1) / d agg1)
|
|
metric_variance = (
|
|
tf.reshape(metric_prime, shape=[1, -1])
|
|
@ N_covariance_estimate
|
|
@ tf.reshape(metric_prime, shape=[-1, 1])
|
|
)
|
|
# result should be a single element, but the matmul is 2D
|
|
metric_variance = metric_variance[0][0]
|
|
metric_stderr = tf.sqrt(metric_variance)
|
|
return metric_stderr
|
|
|
|
metric_stderr = compute_output(aggregates_1, aggregates_2, sample_count)
|
|
update_metric_stderr = compute_output(update_aggregates_1, update_aggregates_2, update_sample_count)
|
|
|
|
if metrics_collections:
|
|
tf.add_to_collections(metrics_collections, metric_stderr)
|
|
|
|
if updates_collections:
|
|
tf.add_to_collections(updates_collections, update_metric_stderr)
|
|
|
|
return metric_stderr, update_metric_stderr
|
|
|
|
|
|
def lolly_nrce(labels, predictions,
|
|
weights=None,
|
|
metrics_collections=None,
|
|
updates_collections=None,
|
|
name=None):
|
|
"""
|
|
Compute the Lolly NRCE.
|
|
|
|
Note: As this NRCE calculation uses Taylor expansion, it becomes inaccurate when the ctr is large,
|
|
especially when the adjusted ctr goes above 1.0.
|
|
|
|
Calculation:
|
|
|
|
::
|
|
|
|
NRCE: lolly NRCE
|
|
BCE: baseline cross entropy
|
|
NCE: normalized cross entropy
|
|
CE: cross entropy
|
|
y_i: label of example i
|
|
p_i: prediction of example i
|
|
y: ctr
|
|
p: average prediction
|
|
a: normalizer
|
|
|
|
Assumes any p_i and a * p_i is within [0, 1)
|
|
NRCE = (1 - NCE / BCE) * 100
|
|
BCE = - sum_i(y_i * log(y) + (1 - y_i) * log(1 - y))
|
|
= - (y * log(y) + (1 - y) * log(1 - y))
|
|
a = y / p
|
|
CE = - sum_i(y_i * log(p_i) + (1 - y_i) * log(1 - p_i))
|
|
NCE = - sum_i(y_i * log(a * p_i) + (1 - y_i) * log(1 - a * p_i))
|
|
= - sum_i(y_i * log(p_i) + (1 - y_i) * log(1 - p_i))
|
|
- sum_i(y_i * log(a))
|
|
+ sum_i((1 - y_i) * log(1 - p_i))
|
|
- sum_i((1 - y_i) * log(1 - a * p_i))
|
|
~= CE - sum_i(y_i) * log(a)
|
|
+ sum_i((1 - y_i) * (- sum_{j=1~5}(p_i^j / j)))
|
|
- sum_i((1 - y_i) * (- sum_{j=1~5}(a^j * p_i^j / j)))
|
|
# Takes 5 items from the Taylor expansion, can be increased if needed
|
|
# Error for each example is O(p_i^6)
|
|
= CE - sum_i(y_i) * log(a)
|
|
- sum_{j=1~5}(sum_i((1 - y_i) * p_i^j) / j)
|
|
+ sum_{j=1~5}(sum_i((1 - y_i) * p_i^j) * a^j / j)
|
|
= CE - sum_i(y_i) * log(a)
|
|
+ sum_{j=1~5}(sum_i((1 - y_i) * p_i^j) * (a^j - 1) / j)
|
|
|
|
Thus we keep track of CE, sum_i(y_i), sum_i((1 - y_i) * p_i^j) for j=1~5.
|
|
We also keep track of p and y by sum_i(y_i), sum_i(p_i), sum_i(1) so that
|
|
we can get a at the end, which leads to this NRCE.
|
|
|
|
NRCE uses ctr and average pctr to normalize the pctrs.
|
|
It removes the impact of prediction error from RCE.
|
|
Usually NRCE is higher as the prediction error impact on RCE is negative.
|
|
Removing prediction error in our model can make RCE closer to NRCE and thus improve RCE.
|
|
|
|
In Lolly NRCE we use ctr and average pctr of the whole dataset.
|
|
We thus remove the dataset level error in NRCE calculation.
|
|
In this case, when we want to improve RCE to the level of NRCE,
|
|
it is achievable as dataset level prediction error is easy to remove by calibration.
|
|
Lolly NRCE is thus a good estimate about the potential gain by adding calibration.
|
|
|
|
In DBv2 NRCE, we use per-batch ctr and average pctr. We remove the batch level error.
|
|
This error is difficult to remove by modeling improvement,
|
|
at least not by simple calibration.
|
|
It thus cannot indicate the same opportunity as the Lolly NRCE does.
|
|
|
|
Args:
|
|
labels:
|
|
the ground true value.
|
|
predictions:
|
|
the predicted values, whose shape must match labels.
|
|
weights:
|
|
optional weights, whose shape must match labels . Weight is 1 if not set.
|
|
metrics_collections:
|
|
optional list of collections to add this metric into.
|
|
updates_collections:
|
|
optional list of collections to add the associated update_op into.
|
|
name:
|
|
an optional variable_scope name.
|
|
|
|
Return:
|
|
rce_value:
|
|
A ``Tensor`` representing the RCE.
|
|
update_op:
|
|
A update operation used to accumulate data into this metric.
|
|
|
|
Note: Must have at least 1 positive and 1 negative sample accumulated,
|
|
or NRCE will come out as NaN.
|
|
"""
|
|
with tf.variable_scope(name, "lolly_nrce", (labels, predictions, weights)):
|
|
labels = tf.to_float(labels, name="label_to_float")
|
|
predictions = tf.to_float(predictions, name="predictions_to_float")
|
|
|
|
if weights is None:
|
|
weights = tf.ones(shape=tf.shape(labels), dtype=tf.float32, name="default_weight")
|
|
else:
|
|
weights = tf.to_float(weights, name="weight_to_float")
|
|
|
|
positive_weights = tf.multiply(labels, weights, name="positive_weights")
|
|
|
|
# clamp predictions to keep log(p) stable
|
|
clip_predictions = tf.clip_by_value(
|
|
predictions,
|
|
CLAMP_EPSILON,
|
|
1.0 - CLAMP_EPSILON,
|
|
name="clip_predictions")
|
|
weighted_predictions = tf.multiply(
|
|
predictions, weights,
|
|
name="weighted_predictions")
|
|
|
|
logloss = _binary_cross_entropy(pred=clip_predictions, target=labels, name="logloss")
|
|
weighted_logloss = tf.multiply(logloss, weights, name="weighted_logloss")
|
|
|
|
negatives = tf.subtract(
|
|
tf.ones(shape=tf.shape(labels), dtype=tf.float32),
|
|
labels,
|
|
name="negatives")
|
|
negative_predictions = tf.multiply(
|
|
predictions,
|
|
negatives,
|
|
name="negative_predictions")
|
|
weighted_negative_predictions = tf.multiply(
|
|
negative_predictions, weights,
|
|
name="weighted_negative_predictions")
|
|
negative_squared_predictions = tf.multiply(
|
|
negative_predictions,
|
|
negative_predictions,
|
|
name="negative_squared_predictions")
|
|
weighted_negative_squared_predictions = tf.multiply(
|
|
negative_squared_predictions, weights,
|
|
name="weighted_negative_squared_predictions")
|
|
negative_cubed_predictions = tf.multiply(
|
|
negative_squared_predictions,
|
|
negative_predictions,
|
|
name="negative_cubed_predictions")
|
|
weighted_negative_cubed_predictions = tf.multiply(
|
|
negative_cubed_predictions, weights,
|
|
name="weighted_negative_cubed_predictions")
|
|
negative_quartic_predictions = tf.multiply(
|
|
negative_cubed_predictions,
|
|
negative_predictions,
|
|
name="negative_quartic_predictions")
|
|
weighted_negative_quartic_predictions = tf.multiply(
|
|
negative_quartic_predictions, weights,
|
|
name="weighted_negative_quartic_predictions")
|
|
negative_quintic_predictions = tf.multiply(
|
|
negative_quartic_predictions,
|
|
negative_predictions,
|
|
name="negative_quintic_predictions")
|
|
weighted_negative_quintic_predictions = tf.multiply(
|
|
negative_quintic_predictions, weights,
|
|
name="weighted_negative_quintic_predictions")
|
|
|
|
# Tracked stats
|
|
total_positive = _metric_variable(name="total_positive", shape=[], dtype=tf.float32)
|
|
total_weight = _metric_variable(name="total_weight", shape=[], dtype=tf.float32)
|
|
|
|
total_prediction = _metric_variable(name="total_prediction", shape=[], dtype=tf.float32)
|
|
|
|
total_negative_prediction = _metric_variable(
|
|
name="total_negative_prediction",
|
|
shape=[], dtype=tf.float32)
|
|
total_negative_squared_prediction = _metric_variable(
|
|
name="total_negative_squared_prediction",
|
|
shape=[], dtype=tf.float32)
|
|
total_negative_cubed_prediction = _metric_variable(
|
|
name="total_negative_cubed_prediction",
|
|
shape=[], dtype=tf.float32)
|
|
total_negative_quartic_prediction = _metric_variable(
|
|
name="total_negative_quartic_prediction",
|
|
shape=[], dtype=tf.float32)
|
|
total_negative_quintic_prediction = _metric_variable(
|
|
name="total_negative_quintic_prediction",
|
|
shape=[], dtype=tf.float32)
|
|
|
|
total_loss = _metric_variable(name="total_loss", shape=[], dtype=tf.float32)
|
|
|
|
# Update tracked stats
|
|
update_total_positive = tf.assign_add(
|
|
total_positive, tf.reduce_sum(positive_weights), name="total_positive_update")
|
|
update_total_weight = tf.assign_add(
|
|
total_weight, tf.reduce_sum(weights), name="total_weight_update")
|
|
update_total_prediction = tf.assign_add(
|
|
total_prediction, tf.reduce_sum(weighted_predictions), name="total_prediction_update")
|
|
update_total_negative_prediction = tf.assign_add(
|
|
total_negative_prediction,
|
|
tf.reduce_sum(weighted_negative_predictions), name="total_negative_prediction_update")
|
|
update_total_negative_squared_prediction = tf.assign_add(
|
|
total_negative_squared_prediction,
|
|
tf.reduce_sum(weighted_negative_squared_predictions),
|
|
name="total_negative_squared_prediction_update")
|
|
update_total_negative_cubed_prediction = tf.assign_add(
|
|
total_negative_cubed_prediction,
|
|
tf.reduce_sum(weighted_negative_cubed_predictions),
|
|
name="total_negative_cubed_prediction_update")
|
|
update_total_negative_quartic_prediction = tf.assign_add(
|
|
total_negative_quartic_prediction,
|
|
tf.reduce_sum(weighted_negative_quartic_predictions),
|
|
name="total_negative_quartic_prediction_update")
|
|
update_total_negative_quintic_prediction = tf.assign_add(
|
|
total_negative_quintic_prediction,
|
|
tf.reduce_sum(weighted_negative_quintic_predictions),
|
|
name="total_negative_quintic_prediction_update")
|
|
update_total_loss = tf.assign_add(
|
|
total_loss, tf.reduce_sum(weighted_logloss), name="total_loss_update")
|
|
|
|
# metric value retrieval subgraph
|
|
# ctr of this batch
|
|
positive_rate = tf.truediv(total_positive, total_weight, name="positive_rate")
|
|
# Note: we don't have to keep running averages for computing baseline CE. Because the prediction
|
|
# is constant for every sample, we can simplify it to the formula below.
|
|
baseline_loss = _binary_cross_entropy(
|
|
pred=positive_rate,
|
|
target=positive_rate,
|
|
name="baseline_loss")
|
|
|
|
# normalizing ratio for nrce
|
|
# calculated using total ctr and pctr so the last batch has the dataset ctr and pctr
|
|
normalizer = tf.truediv(total_positive, total_prediction, name="normalizer")
|
|
# Taylor expansion to calculate nl = - sum(y * log(p * a) + (1 - y) * log (1 - p * a))
|
|
# log(1 - p * a) = -sum_{i=1~+inf}(a^i * x^i / i)
|
|
# log(1 - p) = -sum_{i=1~+inf}(a^i * x^i / i)
|
|
normalized_loss = (
|
|
total_loss -
|
|
total_positive * tf.log(normalizer) +
|
|
total_negative_prediction * (normalizer - 1) +
|
|
total_negative_squared_prediction * (normalizer * normalizer - 1) / 2 +
|
|
total_negative_cubed_prediction *
|
|
(normalizer * normalizer * normalizer - 1) / 3 +
|
|
total_negative_quartic_prediction *
|
|
(normalizer * normalizer * normalizer * normalizer - 1) / 4 +
|
|
total_negative_quintic_prediction *
|
|
(normalizer * normalizer * normalizer * normalizer * normalizer - 1) / 5)
|
|
|
|
# average normalized loss
|
|
avg_loss = tf.truediv(normalized_loss, total_weight, name="avg_loss")
|
|
|
|
nrce_t = tf.multiply(
|
|
1.0 - tf.truediv(avg_loss, baseline_loss),
|
|
100,
|
|
name="lolly_nrce")
|
|
|
|
# metric update subgraph
|
|
update_positive_rate = tf.truediv(
|
|
update_total_positive,
|
|
update_total_weight,
|
|
name="update_positive_rate")
|
|
# Note: we don't have to keep running averages for computing baseline CE. Because the prediction
|
|
# is constant for every sample, we can simplify it to the formula below.
|
|
update_baseline_loss = _binary_cross_entropy(
|
|
pred=update_positive_rate,
|
|
target=update_positive_rate,
|
|
name="update_baseline_loss")
|
|
|
|
update_normalizer = tf.truediv(
|
|
update_total_positive,
|
|
update_total_prediction,
|
|
name="update_normalizer")
|
|
update_normalized_loss = (
|
|
update_total_loss -
|
|
update_total_positive * tf.log(update_normalizer) +
|
|
update_total_negative_prediction *
|
|
(update_normalizer - 1) +
|
|
update_total_negative_squared_prediction *
|
|
(update_normalizer * update_normalizer - 1) / 2 +
|
|
update_total_negative_cubed_prediction *
|
|
(update_normalizer * update_normalizer * update_normalizer - 1) / 3 +
|
|
update_total_negative_quartic_prediction *
|
|
(update_normalizer * update_normalizer * update_normalizer *
|
|
update_normalizer - 1) / 4 +
|
|
update_total_negative_quintic_prediction *
|
|
(update_normalizer * update_normalizer * update_normalizer *
|
|
update_normalizer * update_normalizer - 1) / 5)
|
|
|
|
update_avg_loss = tf.truediv(
|
|
update_normalized_loss,
|
|
update_total_weight,
|
|
name="update_avg_loss")
|
|
|
|
update_op = tf.multiply(
|
|
1.0 - tf.truediv(update_avg_loss, update_baseline_loss),
|
|
100,
|
|
name="update_op")
|
|
|
|
if metrics_collections:
|
|
tf.add_to_collections(metrics_collections, nrce_t)
|
|
|
|
if updates_collections:
|
|
tf.add_to_collections(updates_collections, update_op)
|
|
|
|
return nrce_t, update_op
|
|
|
|
|
|
def _binary_cross_entropy(pred, target, name):
|
|
return - tf.add(
|
|
target * tf.log(pred),
|
|
(1.0 - target) * tf.log(1.0 - pred),
|
|
name=name)
|
|
|
|
|
|
# Copied from metrics_impl.py with minor modifications.
|
|
# https://github.com/tensorflow/tensorflow/blob/v1.5.0/tensorflow/python/ops/metrics_impl.py#L39
|
|
def _metric_variable(shape, dtype, validate_shape=True, name=None):
|
|
"""Create variable in `GraphKeys.(LOCAL|METRIC_VARIABLES`) collections."""
|
|
|
|
return tf.Variable(
|
|
lambda: tf.zeros(shape, dtype),
|
|
trainable=False,
|
|
collections=[tf.GraphKeys.LOCAL_VARIABLES, tf.GraphKeys.METRIC_VARIABLES],
|
|
validate_shape=validate_shape,
|
|
name=name)
|
|
|
|
PERCENTILES = np.linspace(0, 1, 101, dtype=np.float32)
|
|
|
|
# metric_name: (metric, requires thresholded output)
|
|
SUPPORTED_BINARY_CLASS_METRICS = {
|
|
# TWML metrics
|
|
'total_weight': (total_weight_metric, False),
|
|
'num_samples': (num_samples_metric, False),
|
|
'rce': (rce, False),
|
|
'rce_std_err': (partial(metric_std_err, transform=rce_transform, metric=rce_metric, name='rce_std_err'), False),
|
|
'nrce': (partial(rce, normalize=True), False),
|
|
'lolly_nrce': (lolly_nrce, False),
|
|
'arce': (partial(rce, normalize=True, arce=True), False),
|
|
'arce_original': (partial(rce, normalize=True, arce=True, up_weight=False), False),
|
|
# CTR measures positive sample ratio. This terminology is inherited from Ads.
|
|
'ctr': (ctr, False),
|
|
# predicted CTR measures predicted positive ratio.
|
|
'predicted_ctr': (predicted_ctr, False),
|
|
'pred_std_dev': (prediction_std_dev, False),
|
|
# thresholded metrics
|
|
'accuracy': (tf.metrics.accuracy, True),
|
|
'precision': (tf.metrics.precision, True),
|
|
'recall': (tf.metrics.recall, True),
|
|
|
|
'false_positives': (tf.metrics.false_positives, True),
|
|
'false_negatives': (tf.metrics.false_negatives, True),
|
|
'true_positives': (tf.metrics.true_positives, True),
|
|
'true_negatives': (tf.metrics.true_negatives, True),
|
|
|
|
'precision_at_percentiles': (partial(tf.metrics.precision_at_thresholds, thresholds=PERCENTILES), False),
|
|
'recall_at_percentiles': (partial(tf.metrics.recall_at_thresholds, thresholds=PERCENTILES), False),
|
|
'false_positives_at_percentiles': (partial(tf.metrics.false_positives_at_thresholds, thresholds=PERCENTILES), False),
|
|
'false_negatives_at_percentiles': (partial(tf.metrics.false_negatives_at_thresholds, thresholds=PERCENTILES), False),
|
|
'true_positives_at_percentiles': (partial(tf.metrics.true_positives_at_thresholds, thresholds=PERCENTILES), False),
|
|
'true_negatives_at_percentiles': (partial(tf.metrics.true_negatives_at_thresholds, thresholds=PERCENTILES), False),
|
|
|
|
# tensorflow metrics
|
|
'roc_auc': (partial(tf.metrics.auc, curve='ROC',
|
|
summation_method='careful_interpolation'), False),
|
|
'pr_auc': (partial(tf.metrics.auc, curve='PR',
|
|
summation_method='careful_interpolation'), False),
|
|
|
|
# tensorboard curves
|
|
'pr_curve': (tb.summary.v1.pr_curve_streaming_op, False),
|
|
|
|
# deprecated metrics
|
|
'deprecated_nrce': (partial(rce, normalize=True, deprecated_rce=True), False),
|
|
'deprecated_arce': (partial(rce, normalize=True, arce=True, deprecated_rce=True), False),
|
|
'deprecated_arce_original': (partial(rce, normalize=True, arce=True,
|
|
up_weight=False, deprecated_rce=True), False)
|
|
}
|
|
|
|
# default metrics provided by get_binary_class_metric_fn
|
|
DEFAULT_BINARY_CLASS_METRICS = ['total_weight', 'num_samples', 'rce', 'rce_std_err',
|
|
'nrce', 'arce', 'ctr', 'predicted_ctr', 'pred_std_dev',
|
|
'accuracy', 'precision', 'recall', 'roc_auc', 'pr_auc']
|
|
|
|
|
|
def get_binary_class_metric_fn(metrics=None):
|
|
"""
|
|
Returns a function having signature:
|
|
|
|
.. code-block:: python
|
|
|
|
def get_eval_metric_ops(graph_output, labels, weights):
|
|
...
|
|
return eval_metric_ops
|
|
|
|
where the returned eval_metric_ops is a dict of common evaluation metric
|
|
Ops for binary classification. See `tf.estimator.EstimatorSpec
|
|
<https://www.tensorflow.org/api_docs/python/tf/estimator/EstimatorSpec>`_
|
|
for a description of eval_metric_ops. The graph_output is a the result
|
|
dict returned by build_graph. Labels and weights are tf.Tensors.
|
|
|
|
The following graph_output keys are recognized:
|
|
output:
|
|
the raw predictions between 0 and 1. Required.
|
|
threshold:
|
|
A value between 0 and 1 used to threshold the output into a hard_output.
|
|
Defaults to 0.5 when threshold and hard_output are missing.
|
|
Either threshold or hard_output can be provided, but not both.
|
|
hard_output:
|
|
A thresholded output. Either threshold or hard_output can be provided, but not both.
|
|
|
|
Args:
|
|
metrics (list of String):
|
|
a list of metrics of interest. E.g. ['ctr', 'accuracy', 'rce']
|
|
Element in the list can be a string from following supported metrics, or can be a tuple
|
|
with three items: metric name, metric function, bool for thresholded output.
|
|
|
|
These metrics are evaluated and reported to tensorboard *during the eval phases only*.
|
|
Supported metrics:
|
|
|
|
- ctr (same as positive sample ratio.)
|
|
- rce (cross entropy loss compared to the baseline model of always predicting ctr)
|
|
- nrce (normalized rce, do not use this one if you do not understand what it is)
|
|
- `arce <http://go/arce>`_ (a more recent proposed improvment over NRCE)
|
|
- arce_original
|
|
- lolly_nrce (NRCE as it is computed in Lolly, with Taylor expansion)
|
|
- pr_auc
|
|
- roc_auc
|
|
- accuracy (percentage of predictions that are correct)
|
|
- precision (true positives) / (true positives + false positives)
|
|
- recall (true positives) / (true positives + false negatives)
|
|
- pr_curve (precision-recall curve)
|
|
- deprecated_arce (ARCE as it was calculated before a stability fix)
|
|
- deprecated_nrce (NRCE as it was calculated before a stability fix)
|
|
|
|
Example of metrics list with mixture of string and tuple:
|
|
metrics = [
|
|
'rce','nrce',
|
|
'roc_auc', # default roc_auc metric
|
|
(
|
|
'roc_auc_500', # give this metric a name
|
|
partial(tf.metrics.auc, curve='ROC', summation_method='careful_interpolation', num_thresholds=500), # the metric fn
|
|
False, # whether the metric requires thresholded output
|
|
)]
|
|
|
|
NOTE: When predicting rare events roc_auc can be underestimated. Increasing num_threshold
|
|
can reduce the underestimation. See go/roc-auc-pitfall for more details.
|
|
|
|
NOTE: accuracy / precision / recall apply to binary classification problems only.
|
|
I.e. a prediction is only considered correct if it matches the label. E.g. if the label
|
|
is 1.0, and the prediction is 0.99, it does not get credit. If you want to use
|
|
precision / recall / accuracy metrics with soft predictions, you'll need to threshold
|
|
your predictions into hard 0/1 labels.
|
|
|
|
When metrics is None (the default), it defaults to:
|
|
[rce, nrce, arce, ctr, predicted_ctr, accuracy, precision, recall, prauc, roc_auc],
|
|
"""
|
|
# pylint: disable=dict-keys-not-iterating
|
|
if metrics is None:
|
|
# remove expensive metrics by default for faster eval
|
|
metrics = list(DEFAULT_BINARY_CLASS_METRICS)
|
|
|
|
def get_eval_metric_ops(graph_output, labels, weights):
|
|
"""
|
|
graph_output:
|
|
dict that is returned by build_graph given input features.
|
|
labels:
|
|
target labels associated to batch.
|
|
weights:
|
|
weights of the samples..
|
|
"""
|
|
|
|
eval_metric_ops = OrderedDict()
|
|
|
|
preds = graph_output['output']
|
|
|
|
threshold = graph_output['threshold'] if 'threshold' in graph_output else 0.5
|
|
|
|
hard_preds = graph_output.get('hard_output')
|
|
if hard_preds is None:
|
|
hard_preds = tf.greater_equal(preds, threshold)
|
|
|
|
# add metrics to eval_metric_ops dict
|
|
for metric in metrics:
|
|
if isinstance(metric, tuple) and len(metric) == 3:
|
|
metric_name, metric_factory, requires_threshold = metric
|
|
metric_name = metric_name.lower()
|
|
elif isinstance(metric, str):
|
|
metric_name = metric.lower() # metric name are case insensitive.
|
|
metric_factory, requires_threshold = SUPPORTED_BINARY_CLASS_METRICS.get(metric_name)
|
|
else:
|
|
raise ValueError("Metric should be either string or tuple of length 3.")
|
|
|
|
if metric_name in eval_metric_ops:
|
|
# avoid adding duplicate metrics.
|
|
continue
|
|
|
|
if metric_factory:
|
|
value_op, update_op = metric_factory(
|
|
labels=labels,
|
|
predictions=(hard_preds if requires_threshold else preds),
|
|
weights=weights, name=metric_name)
|
|
eval_metric_ops[metric_name] = (value_op, update_op)
|
|
else:
|
|
raise ValueError('Cannot find the metric named ' + metric_name)
|
|
|
|
return eval_metric_ops
|
|
|
|
return get_eval_metric_ops
|
|
|
|
|
|
def get_multi_binary_class_metric_fn(metrics, classes=None, class_dim=1):
|
|
"""
|
|
Returns a function having signature:
|
|
|
|
.. code-block:: python
|
|
|
|
def get_eval_metric_ops(graph_output, labels, weights):
|
|
...
|
|
return eval_metric_ops
|
|
|
|
where the returned eval_metric_ops is a dict of common evaluation metric
|
|
Ops for concatenated binary classifications. See `tf.estimator.EstimatorSpec
|
|
<https://www.tensorflow.org/api_docs/python/tf/estimator/EstimatorSpec>`_
|
|
for a description of eval_metric_ops. The graph_output is a the result
|
|
dict returned by build_graph. Labels and weights are tf.Tensors.
|
|
|
|
In multiple binary classification problems, the
|
|
``predictions`` (that is, ``graph_output['output']``)
|
|
are expected to have shape ``batch_size x n_classes``,
|
|
where ``n_classes`` is the number of binary classification.
|
|
Binary classification at output[i] is expected to discriminate between ``classes[i]`` (1)
|
|
and NOT ``classes[i]`` (0). The labels should be of the same shape as ``graph_output``
|
|
with binary values (0 or 1). The weights can be of size ``batch_size`` or
|
|
``batch_size x n_classes``. The ``class_dim`` contain separate probabilities,
|
|
and need to have separate metrics.
|
|
|
|
The following graph_output keys are recognized:
|
|
output:
|
|
the raw predictions between 0 and 1. Required.
|
|
threshold:
|
|
A value between 0 and 1 used to threshold the output into a hard_output.
|
|
Defaults to 0.5 when threshold and hard_output are missing.
|
|
Either threshold or hard_output can be provided, but not both.
|
|
hard_output:
|
|
A thresholded output. Either threshold or hard_output can be provided, but not both.
|
|
|
|
Args:
|
|
metrics (list of Metrics):
|
|
a list of metrics of interest. E.g. ['ctr', 'accuracy', 'rce']
|
|
Element in the list can be a string from following supported metrics, or can be a tuple
|
|
with three items: metric name, metric function, bool for thresholded output.
|
|
|
|
These metrics are evaluated and reported to tensorboard *during the eval phases only*.
|
|
Supported metrics:
|
|
|
|
- ctr (same as positive sample ratio.)
|
|
- rce (cross entropy loss compared to the baseline model of always predicting ctr)
|
|
- nrce (normalized rce, do not use this one if you do not understand what it is)
|
|
- pr_auc
|
|
- roc_auc
|
|
- accuracy (percentage of predictions that are correct)
|
|
- precision (true positives) / (true positives + false positives)
|
|
- recall (true positives) / (true positives + false negatives)
|
|
- pr_curve (precision-recall curve)
|
|
|
|
Example of metrics list with mixture of string and tuple:
|
|
metrics = [
|
|
'rce','nrce',
|
|
'roc_auc', # default roc_auc metric
|
|
(
|
|
'roc_auc_500', # give this metric a name
|
|
partial(tf.metrics.auc, curve='ROC', summation_method='careful_interpolation', num_thresholds=500), # the metric fn
|
|
False, # whether the metric requires thresholded output
|
|
)]
|
|
|
|
NOTE: When prediction on rare events, roc_auc can be underestimated. Increase num_threshold
|
|
can reduce the underestimation. See go/roc-auc-pitfall for more details.
|
|
|
|
NOTE: accuracy / precision / recall apply to binary classification problems only.
|
|
I.e. a prediction is only considered correct if it matches the label. E.g. if the label
|
|
is 1.0, and the prediction is 0.99, it does not get credit. If you want to use
|
|
precision / recall / accuracy metrics with soft predictions, you'll need to threshold
|
|
your predictions into hard 0/1 labels.
|
|
|
|
When metrics is None (the default), it defaults to:
|
|
[rce, nrce, arce, ctr, predicted_ctr, accuracy, precision, recall, prauc, roc_auc],
|
|
|
|
classes (list of strings):
|
|
In case of multiple binary class models, the names for each class or label.
|
|
These are used to display metrics on tensorboard.
|
|
If these are not specified, the index in the class or label dimension is used, and you'll
|
|
get metrics on tensorboard named like: accuracy_0, accuracy_1, etc.
|
|
|
|
class_dim (number):
|
|
Dimension of the classes in predictions. Defaults to 1, that is, batch_size x n_classes.
|
|
"""
|
|
# pylint: disable=invalid-name,dict-keys-not-iterating
|
|
if metrics is None:
|
|
# remove expensive metrics by default for faster eval
|
|
metrics = list(DEFAULT_BINARY_CLASS_METRICS)
|
|
|
|
def get_eval_metric_ops(graph_output, labels, weights):
|
|
"""
|
|
graph_output:
|
|
dict that is returned by build_graph given input features.
|
|
labels:
|
|
target labels associated to batch.
|
|
weights:
|
|
weights of the samples..
|
|
"""
|
|
|
|
eval_metric_ops = OrderedDict()
|
|
|
|
preds = graph_output['output']
|
|
|
|
threshold = graph_output['threshold'] if 'threshold' in graph_output else 0.5
|
|
|
|
hard_preds = graph_output.get('hard_output')
|
|
if hard_preds is None:
|
|
hard_preds = tf.greater_equal(preds, threshold)
|
|
|
|
shape = labels.get_shape()
|
|
# basic sanity check: multi_metric dimension must exist
|
|
assert len(shape) > class_dim, "Dimension specified by class_dim does not exist."
|
|
|
|
num_labels = shape[class_dim]
|
|
# If we are doing multi-class / multi-label metric, the number of classes / labels must
|
|
# be know at graph construction time. This dimension cannot have size None.
|
|
assert num_labels is not None, "The multi-metric dimension cannot be None."
|
|
assert classes is None or len(classes) == num_labels, (
|
|
"Number of classes must match the number of labels")
|
|
|
|
weights_shape = weights.get_shape() if weights is not None else None
|
|
if weights_shape is None:
|
|
num_weights = None
|
|
elif len(weights_shape) > 1:
|
|
num_weights = weights_shape[class_dim]
|
|
else:
|
|
num_weights = 1
|
|
|
|
for i in range(num_labels):
|
|
|
|
# add metrics to eval_metric_ops dict
|
|
for metric in metrics:
|
|
if isinstance(metric, tuple) and len(metric) == 3:
|
|
metric_name, metric_factory, requires_threshold = metric
|
|
metric_name = metric_name.lower()
|
|
elif isinstance(metric, str):
|
|
metric_name = metric.lower() # metric name are case insensitive.
|
|
metric_factory, requires_threshold = SUPPORTED_BINARY_CLASS_METRICS.get(metric_name)
|
|
else:
|
|
raise ValueError("Metric should be either string or tuple of length 3.")
|
|
|
|
class_metric_name = metric_name + "_" + (classes[i] if classes is not None else str(i))
|
|
|
|
if class_metric_name in eval_metric_ops:
|
|
# avoid adding duplicate metrics.
|
|
continue
|
|
|
|
class_labels = tf.gather(labels, indices=[i], axis=class_dim)
|
|
class_preds = tf.gather(preds, indices=[i], axis=class_dim)
|
|
class_hard_preds = tf.gather(hard_preds, indices=[i], axis=class_dim)
|
|
|
|
if num_weights is None:
|
|
class_weights = None
|
|
elif num_weights == num_labels:
|
|
class_weights = tf.gather(weights, indices=[i], axis=class_dim)
|
|
elif num_weights == 1:
|
|
class_weights = weights
|
|
else:
|
|
raise ValueError("num_weights (%d) and num_labels (%d) do not match"
|
|
% (num_weights, num_labels))
|
|
|
|
if metric_factory:
|
|
value_op, update_op = metric_factory(
|
|
labels=class_labels,
|
|
predictions=(class_hard_preds if requires_threshold else class_preds),
|
|
weights=class_weights, name=class_metric_name)
|
|
eval_metric_ops[class_metric_name] = (value_op, update_op)
|
|
else:
|
|
raise ValueError('Cannot find the metric named ' + metric_name)
|
|
|
|
return eval_metric_ops
|
|
|
|
return get_eval_metric_ops
|
|
|
|
|
|
def _get_uncalibrated_metric_fn(calibrated_metric_fn, keep_weight=True):
|
|
"""
|
|
Returns a function having signature:
|
|
|
|
.. code-block:: python
|
|
|
|
def get_eval_metric_ops(graph_output, labels, weights):
|
|
...
|
|
return eval_metric_ops
|
|
|
|
where the returned eval_metric_ops is a dict of common evaluation metric
|
|
Ops with uncalibrated output.
|
|
|
|
The following graph_output keys are recognized:
|
|
uncalibrated_output:
|
|
the uncalibrated raw predictions between 0 and 1. Required.
|
|
output:
|
|
the calibrated predictions between 0 and 1.
|
|
threshold:
|
|
A value between 0 and 1 used to threshold the output into a hard_output.
|
|
Defaults to 0.5 when threshold and hard_output are missing.
|
|
Either threshold or hard_output can be provided, but not both.
|
|
hard_output:
|
|
A thresholded output. Either threshold or hard_output can be provided, but not both.
|
|
|
|
Args:
|
|
calibrated_metric_fn: metrics function with calibration and weight.
|
|
keep_weight: Bool indicating whether we keep weight.
|
|
"""
|
|
metric_scope = 'uncalibrated' if keep_weight else 'unweighted'
|
|
|
|
def get_eval_metric_ops(graph_output, labels, weights):
|
|
"""
|
|
graph_output:
|
|
dict that is returned by build_graph given input features.
|
|
labels:
|
|
target labels associated to batch.
|
|
weights:
|
|
weights of the samples..
|
|
"""
|
|
with tf.variable_scope(metric_scope):
|
|
if 'uncalibrated_output' not in graph_output:
|
|
raise Exception("Missing uncalibrated_output in graph_output!")
|
|
un_calibrated_weights = weights if keep_weight else tf.ones_like(weights)
|
|
uncalibrated_output = {
|
|
'output': graph_output['uncalibrated_output'],
|
|
'threshold': graph_output.get('threshold', 0.5),
|
|
'hard_output': graph_output.get('hard_output'),
|
|
**{k: v for k, v in graph_output.items() if k not in ['output', 'threshold', 'hard_output']}
|
|
}
|
|
|
|
eval_metrics_ops = calibrated_metric_fn(uncalibrated_output, labels, un_calibrated_weights)
|
|
|
|
renamed_metrics_ops = {f'{metric_scope}_{k}': v for k, v in eval_metrics_ops.items()}
|
|
return renamed_metrics_ops
|
|
|
|
return get_eval_metric_ops
|
|
|
|
|
|
def get_multi_binary_class_uncalibrated_metric_fn(
|
|
metrics, classes=None, class_dim=1, keep_weight=True):
|
|
"""
|
|
Returns a function having signature:
|
|
|
|
.. code-block:: python
|
|
|
|
def get_eval_metric_ops(graph_output, labels, weights):
|
|
...
|
|
return eval_metric_ops
|
|
|
|
where the returned eval_metric_ops is a dict of common evaluation metric
|
|
Ops for concatenated binary classifications without calibration.
|
|
|
|
Note: 'uncalibrated_output' is required key in graph_output.
|
|
|
|
The main use case for this function is:
|
|
|
|
1) To calculated roc-auc for rare event.
|
|
Calibrated prediction score for rare events will be concentrated near zero. As a result,
|
|
the roc-auc can be seriously underestimated with current implementation in tf.metric.auc.
|
|
Since roc-auc is invariant against calibration, we can directly use uncalibrated score for roc-auc.
|
|
For more details, please refer to: go/roc-auc-invariance.
|
|
|
|
2) To set keep_weight=False and get unweighted and uncalibrated metrics.
|
|
This is useful to eval how the model is fitted to its actual training data, since
|
|
often time the model is trained without weight.
|
|
|
|
Args:
|
|
metrics (list of String):
|
|
a list of metrics of interest. E.g. ['ctr', 'accuracy', 'rce']
|
|
Element in the list can be a string from supported metrics, or can be a tuple
|
|
with three items: metric name, metric function, bool for thresholded output.
|
|
These metrics are evaluated and reported to tensorboard *during the eval phases only*.
|
|
|
|
When metrics is None (the default), it defaults to:
|
|
[rce, nrce, arce, ctr, predicted_ctr, accuracy, precision, recall, prauc, roc_auc],
|
|
|
|
classes (list of strings):
|
|
In case of multiple binary class models, the names for each class or label.
|
|
These are used to display metrics on tensorboard.
|
|
If these are not specified, the index in the class or label dimension is used, and you'll
|
|
get metrics on tensorboard named like: accuracy_0, accuracy_1, etc.
|
|
|
|
class_dim (number):
|
|
Dimension of the classes in predictions. Defaults to 1, that is, batch_size x n_classes.
|
|
|
|
keep_weight (bool):
|
|
Whether to keep weights for the metric.
|
|
"""
|
|
|
|
calibrated_metric_fn = get_multi_binary_class_metric_fn(
|
|
metrics, classes=classes, class_dim=class_dim)
|
|
return _get_uncalibrated_metric_fn(calibrated_metric_fn, keep_weight=keep_weight)
|
|
|
|
|
|
def combine_metric_fns(*fn_list):
|
|
"""
|
|
Combine multiple metric functions.
|
|
For example, we can combine metrics function generated by
|
|
get_multi_binary_class_metric_fn and get_multi_binary_class_uncalibrated_metric_fn.
|
|
|
|
Args:
|
|
*fn_list: Multiple metric functions to be combined
|
|
|
|
Returns:
|
|
Combined metric function.
|
|
"""
|
|
def combined_metric_ops(*args, **kwargs):
|
|
eval_metric_ops = OrderedDict()
|
|
for fn in fn_list:
|
|
eval_metric_ops.update(fn(*args, **kwargs))
|
|
return eval_metric_ops
|
|
return combined_metric_ops
|