mirror of
https://github.com/twitter/the-algorithm.git
synced 2024-06-01 08:48:46 +02:00
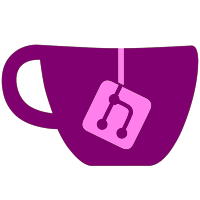
Pushservice is the main recommendation service we use to surface recommendations to our users via notifications. It fetches candidates from various sources, ranks them in order of relevance, and applies filters to determine the best one to send.
166 lines
5.9 KiB
Scala
166 lines
5.9 KiB
Scala
package com.twitter.frigate.pushservice.predicate.quality_model_predicate
|
|
|
|
import com.twitter.finagle.stats.StatsReceiver
|
|
import com.twitter.frigate.pushservice.model.PushTypes
|
|
import com.twitter.frigate.pushservice.model.PushTypes.PushCandidate
|
|
import com.twitter.frigate.pushservice.model.PushTypes.Target
|
|
import com.twitter.frigate.pushservice.target.TargetScoringDetails
|
|
import com.twitter.hermit.predicate.NamedPredicate
|
|
import com.twitter.hermit.predicate.Predicate
|
|
import com.twitter.util.Future
|
|
|
|
object PDauCohort extends Enumeration {
|
|
type PDauCohort = Value
|
|
|
|
val cohort1 = Value
|
|
val cohort2 = Value
|
|
val cohort3 = Value
|
|
val cohort4 = Value
|
|
val cohort5 = Value
|
|
val cohort6 = Value
|
|
}
|
|
|
|
object PDauCohortUtil {
|
|
|
|
case class DauThreshold(
|
|
threshold1: Double,
|
|
threshold2: Double,
|
|
threshold3: Double,
|
|
threshold4: Double,
|
|
threshold5: Double)
|
|
|
|
val defaultDAUProb = 0.0
|
|
|
|
val dauProbThresholds = DauThreshold(
|
|
threshold1 = 0.05,
|
|
threshold2 = 0.14,
|
|
threshold3 = 0.33,
|
|
threshold4 = 0.7,
|
|
threshold5 = 0.959
|
|
)
|
|
|
|
val finerThresholdMap =
|
|
Map(
|
|
PDauCohort.cohort2 -> List(0.05, 0.0539, 0.0563, 0.0600, 0.0681, 0.0733, 0.0800, 0.0849,
|
|
0.0912, 0.0975, 0.1032, 0.1092, 0.1134, 0.1191, 0.1252, 0.1324, 0.14),
|
|
PDauCohort.cohort3 -> List(0.14, 0.1489, 0.1544, 0.1625, 0.1704, 0.1797, 0.1905, 0.2001,
|
|
0.2120, 0.2248, 0.2363, 0.2500, 0.2650, 0.2801, 0.2958, 0.3119, 0.33),
|
|
PDauCohort.cohort4 -> List(0.33, 0.3484, 0.3686, 0.3893, 0.4126, 0.4350, 0.4603, 0.4856,
|
|
0.5092, 0.5348, 0.5602, 0.5850, 0.6087, 0.6319, 0.6548, 0.6779, 0.7),
|
|
PDauCohort.cohort5 -> List(0.7, 0.7295, 0.7581, 0.7831, 0.8049, 0.8251, 0.8444, 0.8612,
|
|
0.8786, 0.8936, 0.9043, 0.9175, 0.9290, 0.9383, 0.9498, 0.9587, 0.959)
|
|
)
|
|
|
|
def getBucket(targetUser: PushTypes.Target, doImpression: Boolean) = {
|
|
implicit val stats = targetUser.stats.scope("PDauCohortUtil")
|
|
if (doImpression) targetUser.getBucket _ else targetUser.getBucketWithoutImpression _
|
|
}
|
|
|
|
def threshold1(targetUser: PushTypes.Target): Double = dauProbThresholds.threshold1
|
|
|
|
def threshold2(targetUser: PushTypes.Target): Double = dauProbThresholds.threshold2
|
|
|
|
def threshold3(targetUser: PushTypes.Target): Double = dauProbThresholds.threshold3
|
|
|
|
def threshold4(targetUser: PushTypes.Target): Double = dauProbThresholds.threshold4
|
|
|
|
def threshold5(targetUser: PushTypes.Target): Double = dauProbThresholds.threshold5
|
|
|
|
def thresholdForCohort(targetUser: PushTypes.Target, dauCohort: Int): Double = {
|
|
if (dauCohort == 0) 0.0
|
|
else if (dauCohort == 1) threshold1(targetUser)
|
|
else if (dauCohort == 2) threshold2(targetUser)
|
|
else if (dauCohort == 3) threshold3(targetUser)
|
|
else if (dauCohort == 4) threshold4(targetUser)
|
|
else if (dauCohort == 5) threshold5(targetUser)
|
|
else 1.0
|
|
}
|
|
|
|
def getPDauCohort(dauProbability: Double, thresholds: DauThreshold): PDauCohort.Value = {
|
|
dauProbability match {
|
|
case dauProb if dauProb >= 0.0 && dauProb < thresholds.threshold1 => PDauCohort.cohort1
|
|
case dauProb if dauProb >= thresholds.threshold1 && dauProb < thresholds.threshold2 =>
|
|
PDauCohort.cohort2
|
|
case dauProb if dauProb >= thresholds.threshold2 && dauProb < thresholds.threshold3 =>
|
|
PDauCohort.cohort3
|
|
case dauProb if dauProb >= thresholds.threshold3 && dauProb < thresholds.threshold4 =>
|
|
PDauCohort.cohort4
|
|
case dauProb if dauProb >= thresholds.threshold4 && dauProb < thresholds.threshold5 =>
|
|
PDauCohort.cohort5
|
|
case dauProb if dauProb >= thresholds.threshold5 && dauProb <= 1.0 => PDauCohort.cohort6
|
|
}
|
|
}
|
|
|
|
def getDauProb(target: TargetScoringDetails): Future[Double] = {
|
|
target.dauProbability.map { dauProb =>
|
|
dauProb.map(_.probability).getOrElse(defaultDAUProb)
|
|
}
|
|
}
|
|
|
|
def getPDauCohort(target: TargetScoringDetails): Future[PDauCohort.Value] = {
|
|
getDauProb(target).map { getPDauCohort(_, dauProbThresholds) }
|
|
}
|
|
|
|
def getPDauCohortWithPDau(target: TargetScoringDetails): Future[(PDauCohort.Value, Double)] = {
|
|
getDauProb(target).map { prob =>
|
|
(getPDauCohort(prob, dauProbThresholds), prob)
|
|
}
|
|
}
|
|
|
|
def updateStats(
|
|
target: PushTypes.Target,
|
|
modelName: String,
|
|
predicateResult: Boolean
|
|
)(
|
|
implicit statsReceiver: StatsReceiver
|
|
): Unit = {
|
|
val dauCohortOp = getPDauCohort(target)
|
|
dauCohortOp.map { dauCohort =>
|
|
val cohortStats = statsReceiver.scope(modelName).scope(dauCohort.toString)
|
|
cohortStats.counter(s"filter_$predicateResult").incr()
|
|
}
|
|
if (target.isNewSignup) {
|
|
val newUserModelStats = statsReceiver.scope(modelName)
|
|
newUserModelStats.counter(s"new_user_filter_$predicateResult").incr()
|
|
}
|
|
}
|
|
}
|
|
|
|
trait QualityPredicateBase {
|
|
def name: String
|
|
def thresholdExtractor: Target => Future[Double]
|
|
def scoreExtractor: PushCandidate => Future[Option[Double]]
|
|
def isPredicateEnabled: PushCandidate => Future[Boolean] = _ => Future.True
|
|
def comparator: (Double, Double) => Boolean =
|
|
(score: Double, threshold: Double) => score >= threshold
|
|
def updateCustomStats(
|
|
candidate: PushCandidate,
|
|
score: Double,
|
|
threshold: Double,
|
|
result: Boolean
|
|
)(
|
|
implicit statsReceiver: StatsReceiver
|
|
): Unit = {}
|
|
|
|
def apply()(implicit statsReceiver: StatsReceiver): NamedPredicate[PushCandidate] = {
|
|
Predicate
|
|
.fromAsync { candidate: PushCandidate =>
|
|
isPredicateEnabled(candidate).flatMap {
|
|
case true =>
|
|
scoreExtractor(candidate).flatMap { scoreOpt =>
|
|
thresholdExtractor(candidate.target).map { threshold =>
|
|
val score = scoreOpt.getOrElse(0.0)
|
|
val result = comparator(score, threshold)
|
|
PDauCohortUtil.updateStats(candidate.target, name, result)
|
|
updateCustomStats(candidate, score, threshold, result)
|
|
result
|
|
}
|
|
}
|
|
case _ => Future.True
|
|
}
|
|
}
|
|
.withStats(statsReceiver.scope(s"predicate_$name"))
|
|
.withName(name)
|
|
}
|
|
}
|