mirror of
https://github.com/twitter/the-algorithm.git
synced 2024-11-16 08:29:21 +01:00
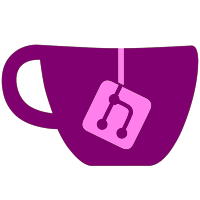
Please note we have force-pushed a new initial commit in order to remove some publicly-available Twitter user information. Note that this process may be required in the future.
562 lines
27 KiB
Python
562 lines
27 KiB
Python
# pylint: disable=protected-access, arguments-differ
|
||
"""
|
||
Command-line argument parsing for the Trainer.
|
||
"""
|
||
import argparse
|
||
from argparse import ArgumentError
|
||
from operator import attrgetter
|
||
import tempfile
|
||
|
||
import twml
|
||
import tensorflow.compat.v1 as tf
|
||
|
||
|
||
SERIAL = "serial"
|
||
TREE = "tree"
|
||
LOG_LEVELS = {
|
||
"debug": tf.logging.DEBUG,
|
||
"info": tf.logging.INFO,
|
||
"warn": tf.logging.WARN,
|
||
"error": tf.logging.ERROR}
|
||
|
||
|
||
class SortingHelpFormatter(argparse.HelpFormatter):
|
||
"""
|
||
Used to sort args alphabetically in the help message.
|
||
"""
|
||
|
||
def add_arguments(self, actions):
|
||
actions = sorted(actions, key=attrgetter('option_strings'))
|
||
super(SortingHelpFormatter, self).add_arguments(actions)
|
||
|
||
|
||
def _set_log_level(level=None):
|
||
"""Sets the tensorflow log level to the input level."""
|
||
if level is None:
|
||
return None
|
||
level = level.lower()
|
||
if level not in LOG_LEVELS.keys():
|
||
raise ValueError(f"Unexpected log level {level} was given but expected one of {LOG_LEVELS.keys()}.")
|
||
tf.logging.set_verbosity(LOG_LEVELS[level])
|
||
tf.logging.info(f"Setting tensorflow logging level to {level} or {LOG_LEVELS[level]}")
|
||
return level
|
||
|
||
|
||
def get_trainer_parser():
|
||
"""
|
||
Add common commandline args to parse for the Trainer class.
|
||
Typically, the user calls this function and then parses cmd-line arguments
|
||
into an argparse.Namespace object which is then passed to the Trainer constructor
|
||
via the params argument.
|
||
|
||
See the `code <_modules/twml/argument_parser.html#get_trainer_parser>`_
|
||
for a list and description of all cmd-line arguments.
|
||
|
||
Args:
|
||
learning_rate_decay:
|
||
Defaults to False. When True, parses learning rate decay arguments.
|
||
|
||
Returns:
|
||
argparse.ArgumentParser instance with some useful args already added.
|
||
"""
|
||
parser = twml.DefaultSubcommandArgParse(formatter_class=SortingHelpFormatter)
|
||
|
||
parser.add_argument(
|
||
"--save_dir", type=str, default=tempfile.mkdtemp(),
|
||
help="Path to the training result directory."
|
||
"supports local filesystem path and hdfs://default/<path> which requires "
|
||
"setting HDFS configuration via env variable HADOOP_CONF_DIR ")
|
||
parser.add_argument(
|
||
"--export_dir", type=str, default=None,
|
||
help="Path to the directory to export a SavedModel for prediction servers.")
|
||
parser.add_argument(
|
||
"--log_aggregation_app_id", type=str, default=None,
|
||
help="specify app_id for log aggregation. disabled by default.")
|
||
parser.add_argument(
|
||
"--train.batch_size", "--train_batch_size", type=int, default=32,
|
||
dest='train_batch_size',
|
||
help="number of samples per training batch")
|
||
parser.add_argument(
|
||
"--eval.batch_size", "--eval_batch_size", type=int, default=32,
|
||
dest='eval_batch_size',
|
||
help="number of samples per cross-validation batch. Defaults to train_batch_size")
|
||
parser.add_argument(
|
||
"--train.learning_rate", "--learning_rate", type=float, default=0.002,
|
||
dest='learning_rate',
|
||
help="learning rate. Scales the gradient update.")
|
||
parser.add_argument(
|
||
"--train.steps", "--train_steps", type=int, default=-1,
|
||
dest='train_steps',
|
||
help="number of training batches before running evaluation."
|
||
"Defaults to -1 (runs through entire dataset). "
|
||
"Only used for Trainer.[train,learn]. "
|
||
"For Trainer.train_and_evaluate, use train.max_steps instead. ")
|
||
parser.add_argument(
|
||
"--eval.steps", "--eval_steps", type=int, default=-1,
|
||
dest="eval_steps",
|
||
help="number of steps per evaluation. Each batch is a step."
|
||
"Defaults to -1 (runs through entire dataset). ")
|
||
parser.add_argument(
|
||
"--eval.period", "--eval_period", type=int, default=600,
|
||
dest="eval_period",
|
||
help="Trainer.train_and_evaluate waits for this long after each evaluation. "
|
||
"Defaults to 600 seconds (evaluate every ten minutes). "
|
||
"Note that anything lower than 10*60seconds is probably a bad idea because TF saves "
|
||
"checkpoints every 10mins by default. eval.delay is time to wait before doing first eval. "
|
||
"eval.period is time between successive evals.")
|
||
parser.add_argument(
|
||
"--eval.delay", "--eval_delay", type=int, default=120,
|
||
dest="eval_delay",
|
||
help="Trainer.train_and_evaluate waits for this long before performing the first evaluation"
|
||
"Defaults to 120 seconds (evaluate after first 2 minutes of training). "
|
||
"eval.delay is time to wait before doing first eval. "
|
||
"eval.period is time between successive evals.")
|
||
parser.add_argument(
|
||
"--train.max_steps", "--train_max_steps", type=int, default=None,
|
||
dest="train_max_steps",
|
||
help="Stop training after this many global steps. Each training batch is its own step."
|
||
"If set to None, step after one train()/evaluate() call. Useful when train.steps=-1."
|
||
"If set to a non-positive value, loop forever. Usually useful with early stopping.")
|
||
parser.add_argument(
|
||
"--train.log_metrics", dest="train_log_metrics", action="store_true", default=False,
|
||
help="Set this to true to see metrics during training. "
|
||
"WARNING: metrics during training does not represent model performance. "
|
||
"WARNING: use for debugging only as this slows down training.")
|
||
parser.add_argument(
|
||
"--train.early_stop_patience", "--early_stop_patience", type=int, default=-1,
|
||
dest="early_stop_patience",
|
||
help="max number of evaluations (epochs) to wait for an improvement in the early_stop_metric."
|
||
"Defaults to -1 (no early-stopping)."
|
||
"NOTE: This can not be enabled when --distributed is also set.")
|
||
parser.add_argument(
|
||
"--train.early_stop_tolerance", "--early_stop_tolerance", type=float, default=0,
|
||
dest="early_stop_tolerance",
|
||
help="a non-negative tolerance for comparing early_stop_metric."
|
||
"e.g. when maximizing the condition is current_metric > best_metric + tolerance."
|
||
"Defaults to 0.")
|
||
parser.add_argument(
|
||
"--train.dataset_shards", "--train_dataset_shards",
|
||
dest="train_dataset_shards",
|
||
type=int, default=None,
|
||
help="An int value that indicates the number of partitions (shards) for the dataset. This is"
|
||
" useful for codistillation and other techniques that require each worker to train on disjoint"
|
||
" partitions of the dataset.")
|
||
parser.add_argument(
|
||
"--train.dataset_shard_index", "--train_dataset_shard_index",
|
||
dest="train_dataset_shard_index",
|
||
type=int, default=None,
|
||
help="An int value (starting at zero) that indicates which partition (shard) of the dataset"
|
||
" to use if --train.dataset_shards is set.")
|
||
parser.add_argument(
|
||
"--continue_from_checkpoint", dest="continue_from_checkpoint", action="store_true",
|
||
help="DEPRECATED. This option is currently a no-op."
|
||
" Continuing from the provided checkpoint is now the default."
|
||
" Use --overwrite_save_dir if you would like to override it instead"
|
||
" and restart training from scratch.")
|
||
parser.add_argument(
|
||
"--overwrite_save_dir", dest="overwrite_save_dir", action="store_true",
|
||
help="Delete the contents of the current save_dir if it exists")
|
||
parser.add_argument(
|
||
"--data_threads", "--num_threads", type=int, default=2,
|
||
dest="num_threads",
|
||
help="Number of threads to use for loading the dataset. "
|
||
"num_threads is deprecated and to be removed in future versions. Use data_threads.")
|
||
parser.add_argument(
|
||
"--max_duration", "--max_duration", type=float, default=None,
|
||
dest="max_duration",
|
||
help="Maximum duration (in secs) that training/validation will be allowed to run for before being automatically terminated.")
|
||
parser.add_argument(
|
||
"--num_workers", type=int, default=None,
|
||
help="Number of workers to use when training in hogwild manner on a single node.")
|
||
parser.add_argument(
|
||
"--distributed", dest="distributed", action="store_true",
|
||
help="Pass this flag to use train_and_evaluate to train in a distributed fashion"
|
||
"NOTE: You can not use early stopping when --distributed is enabled"
|
||
)
|
||
parser.add_argument(
|
||
"--distributed_training_cleanup",
|
||
dest="distributed_training_cleanup",
|
||
action="store_true",
|
||
help="Set if using distributed training on GKE to stop TwitterSetDeployment"
|
||
"from continuing training upon restarts (will be deprecated once we migrate off"
|
||
"TwitterSetDeployment for distributed training on GKE)."
|
||
)
|
||
parser.add_argument(
|
||
"--disable_auto_ps_shutdown", default=False, action="store_true",
|
||
help="Disable the functionality of automatically shutting down parameter server after "
|
||
"distributed training complete (either succeed or failed)."
|
||
)
|
||
parser.add_argument(
|
||
"--disable_tensorboard", default=False, action="store_true",
|
||
help="Do not start the TensorBoard server."
|
||
)
|
||
parser.add_argument(
|
||
"--tensorboard_port", type=int, default=None,
|
||
help="Port for tensorboard to run on. Ignored if --disable_tensorboard is set.")
|
||
parser.add_argument(
|
||
"--health_port", type=int, default=None,
|
||
help="Port to listen on for health-related endpoints (e.g. graceful shutdown)."
|
||
"Not user-facing as it is set automatically by the twml_cli."
|
||
)
|
||
parser.add_argument(
|
||
"--stats_port", type=int, default=None,
|
||
help="Port to listen on for stats endpoints"
|
||
)
|
||
parser.add_argument(
|
||
"--experiment_tracking_path",
|
||
dest="experiment_tracking_path",
|
||
type=str, default=None,
|
||
help="The tracking path of this experiment. Format: \
|
||
user_name:project_name:experiment_name:run_name. The path is used to track and display \
|
||
a record of this experiment on ML Dashboard. Note: this embedded experiment tracking is \
|
||
disabled when the deprecated Model Repo TrackRun is used in your model config. ")
|
||
parser.add_argument(
|
||
"--disable_experiment_tracking",
|
||
dest="disable_experiment_tracking",
|
||
action="store_true",
|
||
help="Whether experiment tracking should be disabled.")
|
||
parser.add_argument(
|
||
"--config.save_checkpoints_secs", "--save_checkpoints_secs", type=int, default=600,
|
||
dest='save_checkpoints_secs',
|
||
help="Configures the tf.estimator.RunConfig.save_checkpoints_secs attribute. "
|
||
"Specifies how often checkpoints are saved in seconds. Defaults to 10*60 seconds.")
|
||
parser.add_argument(
|
||
"--config.keep_checkpoint_max", "--keep_checkpoint_max", type=int, default=20,
|
||
dest='keep_checkpoint_max',
|
||
help="Configures the tf.estimator.RunConfig.keep_checkpoint_max attribute. "
|
||
"Specifies how many checkpoints to keep. Defaults to 20.")
|
||
parser.add_argument(
|
||
"--config.tf_random_seed", "--tf_random_seed", type=int, default=None,
|
||
dest='tf_random_seed',
|
||
help="Configures the tf.estimator.RunConfig.tf_random_seed attribute. "
|
||
"Specifies the seed to use. Defaults to None.")
|
||
parser.add_argument(
|
||
"--optimizer", type=str, default='SGD',
|
||
help="Optimizer to use: SGD (Default), Adagrad, Adam, Ftrl, Momentum, RMSProp, LazyAdam, DGC.")
|
||
parser.add_argument(
|
||
"--gradient_noise_scale", type=float, default=None,
|
||
help="adds 0-mean normal noise scaled by this value. Defaults to None.")
|
||
parser.add_argument(
|
||
"--clip_gradients", type=float, default=None,
|
||
help="If specified, a global clipping is applied to prevent "
|
||
"the norm of the gradient to exceed this value. Defaults to None.")
|
||
parser.add_argument(
|
||
"--dgc.density", "--dgc_density", type=float, default=0.1,
|
||
dest="dgc_density",
|
||
help="Specifies gradient density level when using deep gradient compression optimizer."
|
||
"E.g., default value being 0.1 means that only top 10%% most significant rows "
|
||
"(based on absolute value sums) are kept."
|
||
)
|
||
parser.add_argument(
|
||
"--dgc.density_decay", "--dgc_density_decay", type=bool, default=True,
|
||
dest="dgc_density_decay",
|
||
help="Specifies whether to (exponentially) decay the gradient density level when"
|
||
" doing gradient compression. If set 'False', the 'density_decay_steps', "
|
||
"'density_decay_rate' and 'min_density' arguments will be ignored."
|
||
)
|
||
parser.add_argument(
|
||
"--dgc.density_decay_steps", "--dgc_density_decay_steps", type=int, default=10000,
|
||
dest="dgc_density_decay_steps",
|
||
help="Specifies the step interval to perform density decay."
|
||
)
|
||
parser.add_argument(
|
||
"--dgc.density_decay_rate", "--dgc_density_decay_rate", type=float, default=0.5,
|
||
dest="dgc_density_decay_rate",
|
||
help="Specifies the decay rate when perfoming density decay."
|
||
)
|
||
parser.add_argument(
|
||
"--dgc.min_density", "--dgc_min_density", type=float, default=0.1,
|
||
dest="dgc_min_density",
|
||
help="Specifies the minimum density level when perfoming density decay."
|
||
)
|
||
parser.add_argument(
|
||
"--dgc.accumulation", "--dgc_accumulation", type=bool, default=False,
|
||
dest="dgc_accumulation",
|
||
help="Specifies whether to accumulate small gradients when using deep gradient compression "
|
||
"optimizer."
|
||
)
|
||
parser.add_argument(
|
||
"--show_optimizer_summaries", dest="show_optimizer_summaries", action="store_true",
|
||
help="When specified, displays gradients and learning rate in tensorboard."
|
||
"Turning it on has 10-20%% performance hit. Enable for debugging only")
|
||
|
||
parser.add_argument(
|
||
"--num_mkl_threads", dest="num_mkl_threads", default=1, type=int,
|
||
help="Specifies how many threads to use for MKL"
|
||
"inter_op_ parallelism_threds is set to TWML_NUM_CPUS / num_mkl_threads."
|
||
"intra_op_parallelism_threads is set to num_mkl_threads.")
|
||
|
||
parser.add_argument("--verbosity", type=_set_log_level, choices=LOG_LEVELS.keys(), default=None,
|
||
help="Sets log level to a given verbosity.")
|
||
|
||
parser.add_argument(
|
||
"--feature_importance.algorithm", dest="feature_importance_algorithm",
|
||
type=str, default=TREE, choices=[SERIAL, TREE],
|
||
help="""
|
||
There are two algorithms that the module supports, `serial` and `tree`.
|
||
The `serial` algorithm computes feature importances for each feature, and
|
||
the `tree` algorithm groups features by feature name prefix, computes feature
|
||
importances for groups of features, and then only 'zooms-in' on a group when the
|
||
importance is greater than the `--feature_importance.sensitivity` value. The `tree` algorithm
|
||
will usually run faster, but for relatively unimportant features it will only compute an
|
||
upper bound rather than an exact importance value. We suggest that users generally stick
|
||
to the `tree` algorithm, unless if they have a very small number of features or
|
||
near-random model performance.
|
||
""")
|
||
|
||
parser.add_argument(
|
||
"--feature_importance.sensitivity", dest="feature_importance_sensitivity", type=float, default=0.03,
|
||
help="""
|
||
The maximum amount that permuting a feature group can cause the model performance (determined
|
||
by `feature_importance.metric`) to drop before the algorithm decides to not expand the feature
|
||
group. This is only used for the `tree` algorithm.
|
||
""")
|
||
|
||
parser.add_argument(
|
||
"--feature_importance.dont_build_tree", dest="dont_build_tree", action="store_true", default=False,
|
||
help="""
|
||
If True, don't build the feature trie for the tree algorithm and only use the extra_groups
|
||
""")
|
||
|
||
parser.add_argument(
|
||
"--feature_importance.split_feature_group_on_period", dest="split_feature_group_on_period", action="store_true", default=False,
|
||
help="If true, split feature groups by the period rather than the optimal prefix. Only used for the TREE algorithm")
|
||
|
||
parser.add_argument(
|
||
"--feature_importance.example_count", dest="feature_importance_example_count", type=int, default=10000,
|
||
help="""
|
||
The number of examples used to compute feature importance.
|
||
Larger values yield more reliable results, but also take longer to compute.
|
||
These records are loaded into memory. This number is agnostic to batch size.
|
||
""")
|
||
|
||
parser.add_argument(
|
||
"--feature_importance.data_dir", dest="feature_importance_data_dir", type=str, default=None,
|
||
help="Path to the dataset used to compute feature importance."
|
||
"supports local filesystem path and hdfs://default/<path> which requires "
|
||
"setting HDFS configuration via env variable HADOOP_CONF_DIR "
|
||
"Defaults to eval_data_dir")
|
||
|
||
parser.add_argument(
|
||
"--feature_importance.metric", dest="feature_importance_metric", type=str, default="roc_auc",
|
||
help="The metric used to determine when to stop expanding the feature importance tree. This is only used for the `tree` algorithm.")
|
||
|
||
parser.add_argument(
|
||
"--feature_importance.is_metric_larger_the_better", dest="feature_importance_is_metric_larger_the_better", action="store_true", default=False,
|
||
help="If true, interpret `--feature_importance.metric` to be a metric where larger values are better (e.g. ROC_AUC)")
|
||
|
||
parser.add_argument(
|
||
"--feature_importance.is_metric_smaller_the_better", dest="feature_importance_is_metric_smaller_the_better", action="store_true", default=False,
|
||
help="If true, interpret `--feature_importance.metric` to be a metric where smaller values are better (e.g. LOSS)")
|
||
|
||
subparsers = parser.add_subparsers(help='Learning Rate Decay Functions. Can only pass 1.'
|
||
'Should be specified after all the optional arguments'
|
||
'and followed by its specific args'
|
||
'e.g. --learning_rate 0.01 inverse_learning_rate_decay_fn'
|
||
' --decay_rate 0.0004 --min_learning_rate 0.001',
|
||
dest='learning_rate_decay')
|
||
|
||
# Create the parser for the "exponential_learning_rate_decay_fn"
|
||
parser_exponential = subparsers.add_parser('exponential_learning_rate_decay',
|
||
help='Exponential learning rate decay. '
|
||
'Exponential decay implements:'
|
||
'decayed_learning_rate = learning_rate * '
|
||
'exponential_decay_rate ^ '
|
||
'(global_step / decay_steps')
|
||
parser_exponential.add_argument(
|
||
"--decay_steps", type=float, default=None,
|
||
help="Required for 'exponential' learning_rate_decay.")
|
||
parser_exponential.add_argument(
|
||
"--exponential_decay_rate", type=float, default=None,
|
||
help="Required for 'exponential' learning_rate_decay. Must be positive. ")
|
||
|
||
# Create the parser for the "polynomial_learning_rate_decay_fn"
|
||
parser_polynomial = subparsers.add_parser('polynomial_learning_rate_decay',
|
||
help='Polynomial learning rate decay. '
|
||
'Polynomial decay implements: '
|
||
'global_step = min(global_step, decay_steps)'
|
||
'decayed_learning_rate = '
|
||
'(learning_rate - end_learning_rate) * '
|
||
'(1 - global_step / decay_steps) ^ '
|
||
'(polynomial_power) + end_learning_rate'
|
||
'So for linear decay you can use a '
|
||
'polynomial_power=1 (the default)')
|
||
parser_polynomial.add_argument(
|
||
"--end_learning_rate", type=float, default=0.0001,
|
||
help="Required for 'polynomial' learning_rate_decay (ignored otherwise).")
|
||
parser_polynomial.add_argument(
|
||
"--polynomial_power", type=float, default=0.0001,
|
||
help="Required for 'polynomial' learning_rate_decay."
|
||
"The power of the polynomial. Defaults to linear, 1.0.")
|
||
parser_polynomial.add_argument(
|
||
"--decay_steps", type=float, default=None,
|
||
help="Required for 'polynomial' learning_rate_decay. ")
|
||
|
||
# Create the parser for the "piecewise_constant_learning_rate_decay_fn"
|
||
parser_piecewise_constant = subparsers.add_parser('piecewise_constant_learning_rate_decay',
|
||
help='Piecewise Constant '
|
||
'learning rate decay. '
|
||
'For piecewise_constant, '
|
||
'consider this example: '
|
||
'We want to use a learning rate '
|
||
'that is 1.0 for'
|
||
'the first 100000 steps,'
|
||
'0.5 for steps 100001 to 110000, '
|
||
'and 0.1 for any additional steps. '
|
||
'To do so, specify '
|
||
'--piecewise_constant_boundaries=100000,110000'
|
||
'--piecewise_constant_values=1.0,0.5,0.1')
|
||
parser_piecewise_constant.add_argument(
|
||
"--piecewise_constant_values",
|
||
action=parse_comma_separated_list(element_type=float),
|
||
default=None,
|
||
help="Required for 'piecewise_constant_values' learning_rate_decay. "
|
||
"A list of comma seperated floats or ints that specifies the values "
|
||
"for the intervals defined by boundaries. It should have one more "
|
||
"element than boundaries.")
|
||
parser_piecewise_constant.add_argument(
|
||
"--piecewise_constant_boundaries",
|
||
action=parse_comma_separated_list(element_type=int),
|
||
default=None,
|
||
help="Required for 'piecewise_constant_values' learning_rate_decay. "
|
||
"A list of comma seperated integers, with strictly increasing entries.")
|
||
|
||
# Create the parser for the "inverse_learning_rate_decay_fn"
|
||
parser_inverse = subparsers.add_parser('inverse_learning_rate_decay',
|
||
help='Inverse Leaning rate decay. '
|
||
'Inverse implements:'
|
||
'decayed_lr = max(lr /(1 + decay_rate * '
|
||
'floor(global_step /decay_step)),'
|
||
' min_learning_rate)'
|
||
'When decay_step=1 this mimics the behaviour'
|
||
'of the default learning rate decay'
|
||
'of DeepBird v1.')
|
||
|
||
parser_inverse.add_argument(
|
||
"--decay_rate", type=float, default=None,
|
||
help="Required for 'inverse' learning_rate_decay. Rate in which we decay the learning rate.")
|
||
parser_inverse.add_argument(
|
||
"--min_learning_rate", type=float, default=None,
|
||
help="Required for 'inverse' learning_rate_decay.Minimum possible learning_rate.")
|
||
parser_inverse.add_argument(
|
||
"--decay_steps", type=float, default=1,
|
||
help="Required for 'inverse' learning_rate_decay.")
|
||
|
||
# Create the parser for the "cosine_learning_rate_decay_fn"
|
||
parser_cosine = subparsers.add_parser('cosine_learning_rate_decay',
|
||
help='Cosine Leaning rate decay. '
|
||
'Cosine implements:'
|
||
'decayed_lr = 0.5 * (1 + cos(pi *\
|
||
global_step / decay_steps)) * lr'
|
||
)
|
||
|
||
parser_cosine.add_argument(
|
||
"--alpha", type=float, default=0,
|
||
help="A scalar float32 or float64 Tensor or a Python number.\
|
||
Minimum learning rate value as a fraction of learning_rate.")
|
||
parser_cosine.add_argument(
|
||
"--decay_steps", type=float,
|
||
help="Required for 'inverse' learning_rate_decay.")
|
||
|
||
# Create the parser for the "cosine_restart_learning_rate_decay_fn"
|
||
parser_cosine_restart = subparsers.add_parser('cosine_restarts_learning_rate_decay',
|
||
help='Applies cosine decay with restarts \
|
||
to the learning rate'
|
||
'See [Loshchilov & Hutter, ICLR2016],\
|
||
SGDR: Stochastic'
|
||
'Gradient Descent with Warm Restarts.'
|
||
'https://arxiv.org/abs/1608.03983'
|
||
)
|
||
parser_cosine_restart.add_argument(
|
||
"--first_decay_steps", type=float,
|
||
help="Required for 'cosine_restart' learning_rate_decay.")
|
||
parser_cosine_restart.add_argument(
|
||
"--alpha", type=float, default=0,
|
||
help="A scalar float32 or float64 Tensor or a Python number. \
|
||
Minimum learning rate value as a fraction of learning_rate.")
|
||
parser_cosine_restart.add_argument(
|
||
"--t_mul", type=float, default=2,
|
||
help="A scalar float32 or float64 Tensor or a Python number. \
|
||
Used to derive the number of iterations in the i-th period")
|
||
parser_cosine_restart.add_argument(
|
||
"--m_mul", type=float, default=1,
|
||
help="A scalar float32 or float64 Tensor or a Python number. \
|
||
Used to derive the initial learning rate of the i-th period.")
|
||
|
||
# Create dummy parser for None, which is the default.
|
||
parser_default = subparsers.add_parser(
|
||
'no_learning_rate_decay',
|
||
help='No learning rate decay') # noqa: F841
|
||
|
||
parser.set_default_subparser('no_learning_rate_decay')
|
||
|
||
return parser
|
||
|
||
|
||
class DefaultSubcommandArgParse(argparse.ArgumentParser):
|
||
"""
|
||
Subclass of argparse.ArgumentParser that sets default parser
|
||
"""
|
||
_DEFAULT_SUBPARSER = None
|
||
|
||
def set_default_subparser(self, name):
|
||
"""
|
||
sets the default subparser
|
||
"""
|
||
self._DEFAULT_SUBPARSER = name
|
||
|
||
def _parse_known_args(self, arg_strings, *args, **kwargs):
|
||
"""
|
||
Overwrites _parse_known_args
|
||
"""
|
||
in_args = set(arg_strings)
|
||
d_sp = self._DEFAULT_SUBPARSER
|
||
if d_sp is not None and not {'-h', '--help'}.intersection(in_args):
|
||
for x_val in self._subparsers._actions:
|
||
subparser_found = (
|
||
isinstance(x_val, argparse._SubParsersAction) and
|
||
in_args.intersection(x_val._name_parser_map.keys())
|
||
)
|
||
if subparser_found:
|
||
break
|
||
else:
|
||
# insert default in first position, this implies no
|
||
# global options without a sub_parsers specified
|
||
arg_strings = arg_strings + [d_sp]
|
||
return super(DefaultSubcommandArgParse, self)._parse_known_args(
|
||
arg_strings, *args, **kwargs
|
||
)
|
||
|
||
def _check_value(self, action, value):
|
||
try:
|
||
super(DefaultSubcommandArgParse, self)._check_value(
|
||
action, value
|
||
)
|
||
except ArgumentError as error:
|
||
error.message += ("\nERROR: Deepbird is trying to interpret \"{}\" as a value of {}. If this is not what you expected, "
|
||
"then most likely one of the following two things are happening: Either one of your cli arguments are not recognized, "
|
||
"probably {} or whichever argument you are passing {} as a value to OR you are passing in an argument after "
|
||
"the `learning_rate_decay` argument.\n").format(value, action.dest, value, value)
|
||
raise error
|
||
|
||
|
||
def parse_comma_separated_list(element_type=str):
|
||
"""
|
||
Generates an argparse.Action that converts a string representing a comma separated list to a
|
||
list and converts each element to a specified type.
|
||
"""
|
||
|
||
# pylint: disable-msg=too-few-public-methods
|
||
class _ParseCommaSeparatedList(argparse.Action):
|
||
"""
|
||
Converts a string representing a comma separated list to a list and converts each element to a
|
||
specified type.
|
||
"""
|
||
|
||
def __call__(self, parser, namespace, values, option_string=None):
|
||
if values is not None:
|
||
values = [element_type(v) for v in values.split(',')]
|
||
setattr(namespace, self.dest, values)
|
||
|
||
return _ParseCommaSeparatedList
|