mirror of
https://github.com/twitter/the-algorithm.git
synced 2024-12-23 10:41:50 +01:00
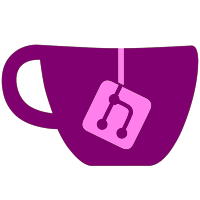
Please note we have force-pushed a new initial commit in order to remove some publicly-available Twitter user information. Note that this process may be required in the future.
373 lines
15 KiB
Python
373 lines
15 KiB
Python
"""
|
|
This module implements custom tf.data.datasets for twml.
|
|
"""
|
|
import numbers
|
|
|
|
from absl import logging
|
|
from kazoo.client import KazooClient
|
|
from libtwml import OPLIB
|
|
import tensorflow.compat.v1 as tf
|
|
from twml.constants import DEFAULT_ZOOKEEPER_BASE_ZNODE, DEFAULT_ZOOKEEPER_HOST
|
|
|
|
|
|
class BlockFormatDataset(tf.data.Dataset):
|
|
"""A ``tf.data.Dataset`` comprising records from one or more TFRecord files."""
|
|
|
|
def __init__(self, filenames, compression_type="auto", buffer_size=1 << 20):
|
|
"""
|
|
Creates a ``BlockFormatDataset``.
|
|
|
|
Args:
|
|
filenames:
|
|
A `tf.string` tensor containing one or more filenames.
|
|
compression_type:
|
|
A string specifying the compression type.
|
|
Can be one of 'gz' (or 'gzip'), 'none', 'auto' (default).
|
|
When compression_type == 'auto', it is inferred from file extension.
|
|
buffer_size:
|
|
Buffer size to be used during decompression. default: 1<<20.
|
|
"""
|
|
self._filenames = tf.convert_to_tensor(filenames, dtype=tf.string, name="filenames")
|
|
self._compression_type = tf.convert_to_tensor(compression_type.lower(), name="compression_type")
|
|
self._buffer_size = tf.convert_to_tensor(buffer_size, dtype=tf.int64, name="buffer_size")
|
|
# Parent class calss self._as_variant_tensor in init. So call this at the end.
|
|
super(BlockFormatDataset, self).__init__()
|
|
|
|
def _as_variant_tensor(self):
|
|
"""
|
|
Create the resource handle for the dataset.
|
|
"""
|
|
try:
|
|
block_format_dataset = __import__("libtwml_internal").OPLIB.block_format_dataset
|
|
return block_format_dataset(self._filenames)
|
|
except ImportError:
|
|
block_format_dataset = OPLIB.block_format_dataset_v2
|
|
return block_format_dataset(self._filenames, self._compression_type, self._buffer_size)
|
|
|
|
def _inputs(self):
|
|
return []
|
|
|
|
@property
|
|
def output_shapes(self):
|
|
"""Return output shapes"""
|
|
return tf.TensorShape([])
|
|
|
|
@property
|
|
def output_types(self):
|
|
"""Return output types"""
|
|
return tf.string
|
|
|
|
@property
|
|
def output_classes(self):
|
|
"""Return output classes"""
|
|
return tf.Tensor
|
|
|
|
|
|
def downsample_dataset(dataset, sample_rate, rate_name):
|
|
"""
|
|
Downsample a tf.data.Dataset at sample_rate
|
|
"""
|
|
if sample_rate is None or sample_rate == 1.0:
|
|
return dataset
|
|
elif not isinstance(sample_rate, numbers.Real):
|
|
raise TypeError("dataset %s must be a real number" % rate_name)
|
|
elif sample_rate <= 0 or sample_rate > 1:
|
|
raise ValueError("dataset %s must be in range (0, 1])" % rate_name)
|
|
return dataset.filter(lambda _: tf.squeeze(tf.random_uniform([1])) < sample_rate)
|
|
|
|
|
|
def _filenames_dataset(files, shards=None, shard_index=None):
|
|
"""
|
|
Get a tf.data.Dataset with file names from a list of files
|
|
Optionally shard the file list (see stream_block_format_dataset)
|
|
"""
|
|
files = tf.data.Dataset.from_tensor_slices(files)
|
|
|
|
if [shards, shard_index] != [None, None]:
|
|
logging.info("Sharding files dataset (index: %d, shards: %d)" % (shard_index, shards))
|
|
files = files.shard(num_shards=shards, index=shard_index)
|
|
|
|
return files
|
|
|
|
|
|
def stream_block_format_dataset(
|
|
files, parse_fn, batch_size, num_threads,
|
|
shuffle=True, repeat=False,
|
|
block_length=None, part_file_parallelism=None, file_shuffle_size=None,
|
|
record_shuffle_size=None, dataset_fn=None,
|
|
keep_rate=None, parts_downsampling_rate=None, prefetch_size=2,
|
|
shards=None, shard_index=None, shuffle_files=True, interleave=True):
|
|
"""
|
|
Helper function to stream a list of part files.
|
|
|
|
Args:
|
|
files:
|
|
List of input files which will create a dataset.
|
|
parse_fn:
|
|
A function that takes a byte tensor containing a datarecord and decodes it.
|
|
batch_size:
|
|
The batch size for each step.
|
|
num_threads:
|
|
Number of threads working on the data in parallel.
|
|
shuffle:
|
|
Shuffle records within each file using ``record_shuffle_size``. Defaults to True.
|
|
repeat:
|
|
Repeat the dataset indefinitely. Defaults to False.
|
|
Useful when you want to use an ``[train,eval]_steps`` greater than the size of the dataset
|
|
(otherwise ``Estimator.[train,evaluate]`` stop when the end of the dataset is reached).
|
|
block_length (optional):
|
|
Number of consecutive records to pull from a single part file.
|
|
Defaults to batch_size.
|
|
part_file_parallelism (optional):
|
|
Number of part files to read from in parallel. Once a part file is completely read, it will
|
|
be replaced by the next part file in the part file list.
|
|
|
|
``num_threads`` specifies a reader thread pool size, while ``part_file_parallelism`` specifies
|
|
the number of files to read from in parallel. If ``part_file_parallelism`` is greater than or
|
|
equal to ``num_threads``, the reads will be distributed over ``num_threads``. On the other hand,
|
|
if ``part_file_parallelism`` is smaller than``num_threads``, it is very likely that the reader
|
|
thread pool will be underutilized, since it can never be the case that every reader thread has
|
|
a part file to read from.
|
|
|
|
file_shuffle_size (optional):
|
|
the buffer_size used for shuffling of the list of files.
|
|
Defaults to 1000. For example, if you have 2000 files, the first
|
|
1000 files are shuffled together, iterated through, then the next 1000 files are shuffled
|
|
and iterated through.
|
|
record_shuffle_size (optional):
|
|
the ``buffer_size`` used for shuffling records in each thread.
|
|
Defaults to ``batch_size * 8`` records.
|
|
dataset_fn (optional):
|
|
A function of that modifies the dataset after it reads different interleaved parts files.
|
|
Defaults to:
|
|
|
|
.. code-block:: python
|
|
|
|
def dataset_fn(dataset, parse_fn, batch_size):
|
|
return dataset.batch(batch_size).map(parse_fn, 1)
|
|
|
|
keep_rate (optional):
|
|
A float value in (0.0, 1.0] that indicates to drop records according to the Bernoulli
|
|
distribution with p = 1 - keep_rate.
|
|
Defaults to None (no records dropped).
|
|
|
|
parts_downsampling_rate (optional):
|
|
A float value in ``(0.0, 1.0]`` that indicates the factor by which to downsample part files.
|
|
For example, a value of 0.2 means only 20 percent of part files become part of the dataset.
|
|
|
|
Note that this argument is only useful in conjunction with a [train,eval]_steps of -1
|
|
(that is, when the entire dataset is used). Furthermore, note that even in this case, each
|
|
epoch will see a different set of part files. This is because new part files are re-sampled
|
|
every epoch. In other words, this argument is only provided for backwards compatibility with
|
|
DeepBird v1. We recommend you use a smaller [train,eval]_steps (or specify a keep_rate)
|
|
instead.
|
|
|
|
shards (optional):
|
|
Number of partitions to shard the dataset into. This is useful for codistillation and other
|
|
techniques that require each worker to train on disjoint partitions of the dataset.
|
|
The dataset is not sharded by default.
|
|
|
|
shard_index (optional):
|
|
Which partition of the dataset to use if ``shards`` is set.
|
|
|
|
shuffle_files (optional):
|
|
Shuffle the list of files. Defaults to True.
|
|
When False, files are iterated in the order they are passed in.
|
|
|
|
interleave (optional):
|
|
Interleave records from multiple files in parallel. Defaults to True.
|
|
|
|
Returns:
|
|
tf.data.DataSet of batches of HashedDataRecord resource handles decoded and streamed online.
|
|
"""
|
|
# Creating a dataset from an input directory
|
|
|
|
files = _filenames_dataset(files, shards=shards, shard_index=shard_index)
|
|
|
|
file_shuffle_size = file_shuffle_size if file_shuffle_size is not None else 100000
|
|
record_shuffle_size = record_shuffle_size if record_shuffle_size is not None else (batch_size * 8)
|
|
block_length = block_length if block_length is not None else batch_size
|
|
|
|
logging.info("NUM_THREADS: %d", num_threads)
|
|
|
|
if repeat:
|
|
files = files.repeat()
|
|
|
|
if shuffle_files:
|
|
# Randomly shuffle the files list.
|
|
files = files.shuffle(buffer_size=file_shuffle_size)
|
|
|
|
# Downsample parts files
|
|
files = downsample_dataset(files, parts_downsampling_rate, "parts_downsampling_rate")
|
|
|
|
# Interleave the result from BlockFormatDataset
|
|
# block_length == batch_size results in batch_size records being read from a single file.
|
|
def map_fn(filenames):
|
|
'''function that maps each filename to a BlockFormatDataset'''
|
|
# reach each file using BlockFormatDataset
|
|
dataset = BlockFormatDataset(filenames)
|
|
|
|
# early prefetching can sometimes improve performance (like on GCS)
|
|
dataset = dataset.prefetch(tf.data.experimental.AUTOTUNE)
|
|
|
|
# Shuffling before repeating ensures strong ordering.
|
|
if shuffle:
|
|
dataset = dataset.shuffle(buffer_size=record_shuffle_size)
|
|
|
|
return dataset
|
|
|
|
if interleave:
|
|
part_file_parallelism = num_threads if part_file_parallelism is None else part_file_parallelism
|
|
dataset = files.interleave(
|
|
map_fn, cycle_length=part_file_parallelism, block_length=block_length, num_parallel_calls=num_threads)
|
|
else:
|
|
dataset = files.flat_map(map_fn)
|
|
|
|
# Downsample DataRecords
|
|
dataset = downsample_dataset(dataset, keep_rate, "keep_rate")
|
|
|
|
if dataset_fn is None:
|
|
# Create a batch of datarecords and decode them
|
|
return dataset.batch(batch_size).map(parse_fn, num_parallel_calls=tf.data.experimental.AUTOTUNE).prefetch(prefetch_size)
|
|
|
|
return dataset_fn(dataset, parse_fn, batch_size)
|
|
|
|
|
|
def cx_zk_path(path):
|
|
if path is None:
|
|
raise ValueError("Path for zookeeper dataset pointer is None. You must specify a path.")
|
|
return_path = "/".join([DEFAULT_ZOOKEEPER_BASE_ZNODE, path])
|
|
logging.info("Zookeeper path is: {}".format(return_path))
|
|
return return_path
|
|
|
|
|
|
def zookeeper_ordered_dataset(
|
|
files, parse_fn, batch_size, zk_counter_path, repeat=False,
|
|
num_threads=2, block_length=None, part_file_parallelism=None,
|
|
batch_shuffle_size=None, file_keep_rate=None, record_keep_rate=None,
|
|
prefetch_size=2, interleave=False, dataset_fn=None, verbose=False):
|
|
"""
|
|
Make a tf.Dataset given an ordered list of filenames, using Zookeeper to keep track of
|
|
which file to read, and to coordinate multiple workers.
|
|
|
|
Args:
|
|
files:
|
|
ordered list of (typically HDFS) filenames. This must remain consistent
|
|
between different workers, and between worker restarts (e.g. in the case
|
|
of instance failure or preemption).
|
|
To ensure this remains consistent, consider using the --train.files_list
|
|
option from DataRecordTrainer.
|
|
parse_fn:
|
|
A function that takes a byte tensor containing a datarecord and decodes it.
|
|
batch_size:
|
|
The batch size for each step.
|
|
zk_counter_path:
|
|
Path under the root node for the underlying zookeeper shared counter that
|
|
is used to coordinate distributed iteration over the list of files.
|
|
Full path will be `'/'.join([DEFAULT_ZOOKEEPER_BASE_ZNODE, zk_counter_path])`.
|
|
repeat:
|
|
Default False. Set True to repeat over the files forever.
|
|
num_threads:
|
|
Default 2. Number of threads working on the data in parallel.
|
|
Only used if interleave=True.
|
|
block_length:
|
|
Default None. Number of consecutive records to pull from a single part file.
|
|
If None, then block_length=batch_size will be used.
|
|
Only used if interleave=True.
|
|
part_file_parallelism:
|
|
Default None. Number of part files to read from in parallel. Once a part file is completely
|
|
read, it will be replaced by the next part file indicated by the zookeeper counter.
|
|
Only used if interleave=True.
|
|
|
|
``num_threads`` specifies a reader thread pool size, while ``part_file_parallelism`` specifies
|
|
the number of files to read from in parallel. If ``part_file_parallelism`` is greater than or
|
|
equal to ``num_threads``, the reads will be distributed over ``num_threads``. On the other hand,
|
|
if ``part_file_parallelism`` is smaller than``num_threads``, it is very likely that the reader
|
|
thread pool will be underutilized, since it can never be the case that every reader thread has
|
|
a part file to read from.
|
|
|
|
batch_shuffle_size:
|
|
Default None. Size of shuffle buffer, for shuffling that will be applied after batching.
|
|
if None, then batches will not be shuffled. Ignored if dataset_fn is provided.
|
|
file_keep_rate:
|
|
Default None. Fraction of files to keep, or None to keep all files.
|
|
record_keep_rate:
|
|
Default None. Fraction of records to keep, or None to keep all records.
|
|
prefetch_size:
|
|
Default 2. Number of parsed batches to prefetch. Ignored if dataset_fn is provided.
|
|
interleave:
|
|
Default False. Set True to use tf.data.Dataset.interleave rather than flat_map.
|
|
dataset_fn:
|
|
A function that is applied to the dataset of individual records, after
|
|
these have been read from the parts files.
|
|
If ``None`` (the default), the behavior will be as though dataset_fn were set to:
|
|
|
|
.. code-block:: python
|
|
|
|
def dataset_fn(dataset, parse_fn, batch_size):
|
|
dataset = dataset.batch(batch_size)
|
|
dataset = dataset.map(parse_fn, tf.data.experimental.AUTOTUNE)
|
|
if batch_shuffle_size:
|
|
dataset = dataset.shuffle(batch_shuffle_size)
|
|
return dataset.prefetch(prefetch_size)
|
|
|
|
verbose:
|
|
Default False. Set True to log the names of files loaded by TF.
|
|
"""
|
|
block_length = batch_size if block_length is None else block_length
|
|
part_file_parallelism = num_threads if part_file_parallelism is None else part_file_parallelism
|
|
|
|
def zk_index_generator(my_files=files):
|
|
zk = KazooClient(hosts=DEFAULT_ZOOKEEPER_HOST)
|
|
zk.start()
|
|
my_counter = zk.Counter(cx_zk_path(zk_counter_path), default=0)
|
|
while True:
|
|
my_counter += 1
|
|
counter_pre_value = my_counter.pre_value
|
|
if repeat:
|
|
counter_pre_value = counter_pre_value % len(my_files)
|
|
if counter_pre_value >= len(my_files):
|
|
break
|
|
else:
|
|
chosen_file = my_files[counter_pre_value]
|
|
if verbose:
|
|
logging.info("{}. yielding {}".format(counter_pre_value, chosen_file))
|
|
yield chosen_file
|
|
zk.stop()
|
|
|
|
files = tf.data.Dataset.from_generator(zk_index_generator, tf.string)
|
|
|
|
# Downsample parts files
|
|
files = downsample_dataset(files, file_keep_rate, "file_keep_rate")
|
|
|
|
def map_fn(filenames):
|
|
return BlockFormatDataset(filenames).prefetch(20)
|
|
|
|
# Dont interleave for sequential training
|
|
if interleave:
|
|
dataset = files.interleave(
|
|
map_fn,
|
|
cycle_length=part_file_parallelism,
|
|
block_length=block_length,
|
|
num_parallel_calls=num_threads)
|
|
else:
|
|
dataset = files.flat_map(map_fn)
|
|
|
|
# Downsample DataRecords
|
|
dataset = downsample_dataset(dataset, record_keep_rate, "record_keep_rate")
|
|
|
|
if dataset_fn is None:
|
|
# Create a batch of datarecords and decode them
|
|
dataset = dataset.batch(batch_size)
|
|
dataset = dataset.map(parse_fn, num_parallel_calls=tf.data.experimental.AUTOTUNE)
|
|
# shuffle after batching and parsing for performance reasons
|
|
# faster b/c 1 random selection is made per batch rather than per record
|
|
if batch_shuffle_size:
|
|
dataset = dataset.shuffle(buffer_size=batch_shuffle_size)
|
|
dataset = dataset.prefetch(prefetch_size)
|
|
|
|
else:
|
|
dataset = dataset_fn(dataset, parse_fn, batch_size)
|
|
|
|
return dataset
|